Convolution operation structure for reducing data migration and power consumption of deep neural network
A deep neural network and convolution operation technology, applied in the field of convolution operation structure, can solve the problems of single mining reusability, not considering weight reusability, poor flexibility and adaptability of PE array storage structure, etc. Versatility, simple control structure, and the effect of reducing dynamic power consumption
- Summary
- Abstract
- Description
- Claims
- Application Information
AI Technical Summary
Problems solved by technology
Method used
Image
Examples
Embodiment Construction
[0030]The present invention provides a convolution operation structure that reduces the data migration and power consumption of deep neural networks, fully analyzes the current mainstream convolution calculation data multiplexing system, and proposes a data multiplexing system that combines elements such as weights and input feature maps. The main purpose of this scheme is to reduce the number of times different elements move between PE arrays in the space and time dimensions, thereby reducing the number of accesses to low-level memory, thereby effectively reducing the dynamic power consumption of global computing. The present invention provides a qualitative description of the calculation process for the proposed method, and at the same time provides a quantitative evaluation formula for the feature data access compression rate after adopting the method of the present invention to verify the effectiveness of the scheme, and finally provides a specific implementation structure a...
PUM
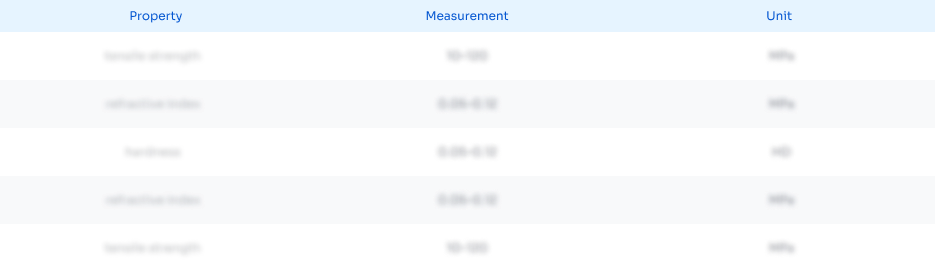
Abstract
Description
Claims
Application Information
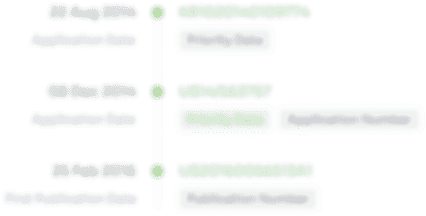
- R&D Engineer
- R&D Manager
- IP Professional
- Industry Leading Data Capabilities
- Powerful AI technology
- Patent DNA Extraction
Browse by: Latest US Patents, China's latest patents, Technical Efficacy Thesaurus, Application Domain, Technology Topic, Popular Technical Reports.
© 2024 PatSnap. All rights reserved.Legal|Privacy policy|Modern Slavery Act Transparency Statement|Sitemap|About US| Contact US: help@patsnap.com