FPGA-based space-time diagram neural network accelerator structure
A neural network and accelerator technology, applied in the field of space-time graph neural network accelerator structure, can solve problems such as difficult performance, difficult to adapt to network models including various computing styles, complex computing features, etc., to achieve efficient processing, strong universality, and simplicity way effect
- Summary
- Abstract
- Description
- Claims
- Application Information
AI Technical Summary
Problems solved by technology
Method used
Image
Examples
Embodiment Construction
[0056] In order to solve the defect that the existing technology can only accelerate for a certain neural network structure, and it is difficult to adapt to the network model containing multiple computing styles, the present invention designs a spatiotemporal graph neural network accelerator structure based on FPGA. The parallel processing method of tensor and vector dual acceleration modules, the vector acceleration module performs sparse matrix multiplication or performs element-wise multiplication and addition operations, and the tensor acceleration module performs dense matrix multiplication, bias term addition, and operations of different activation functions. Moreover, the system control module controls the tensor acceleration module and the vector acceleration module to complete the calculation corresponding to the calculation sequence according to the set calculation sequence, and the data flow control module controls the data between the tensor acceleration module and t...
PUM
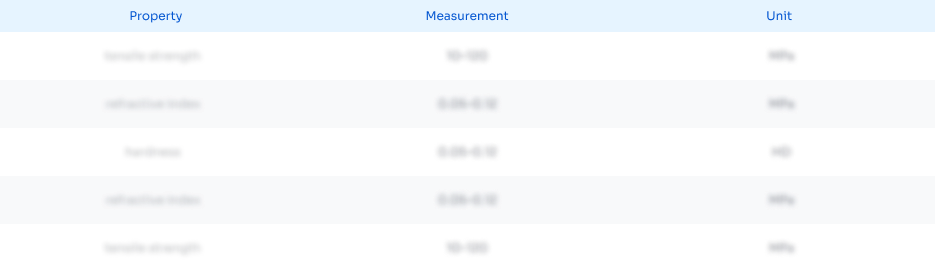
Abstract
Description
Claims
Application Information
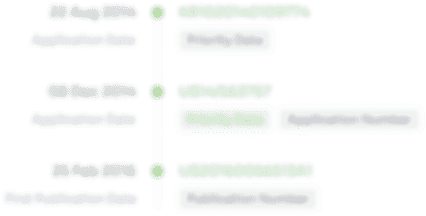
- R&D Engineer
- R&D Manager
- IP Professional
- Industry Leading Data Capabilities
- Powerful AI technology
- Patent DNA Extraction
Browse by: Latest US Patents, China's latest patents, Technical Efficacy Thesaurus, Application Domain, Technology Topic, Popular Technical Reports.
© 2024 PatSnap. All rights reserved.Legal|Privacy policy|Modern Slavery Act Transparency Statement|Sitemap|About US| Contact US: help@patsnap.com