FPGA-based AI chip neural network acceleration method
A neural network and chip technology, applied in the field of neural network acceleration, can solve problems such as excessive volume, low flexibility, and low product migration
- Summary
- Abstract
- Description
- Claims
- Application Information
AI Technical Summary
Problems solved by technology
Method used
Image
Examples
Embodiment Construction
[0049] The present invention provides an FPGA chip-based neural network acceleration method, which can quantify the convolutional neural network and arrange it in an edge AI chip for efficient calculation. At the same time, this method adopts a high-level synthesis method to design the IP core of the convolutional neural network accelerator, which realizes rapid development and design. In the calculation process of convolutional neural network, algorithm design is used to reduce computational complexity and achieve the purpose of accelerating neural network. Under the premise of ensuring the accuracy, the neural network is compressed and accelerated to realize the deployment of artificial intelligence algorithms on embedded devices, which is mainly used in the implementation of AI algorithms in edge scenarios. At the same time, this method utilizes the reconfigurability of FPGA to realize the joint design of software and hardware, which effectively solves the shortcomings of o...
PUM
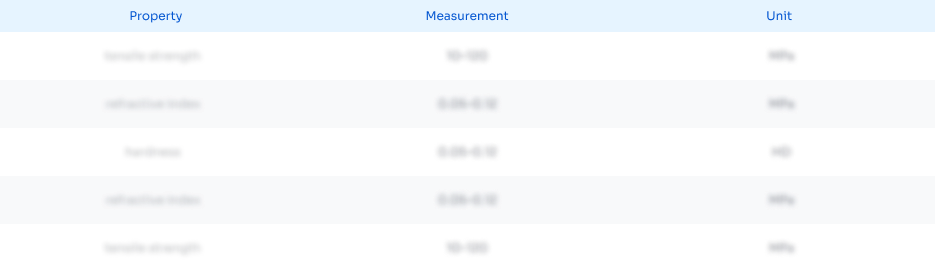
Abstract
Description
Claims
Application Information
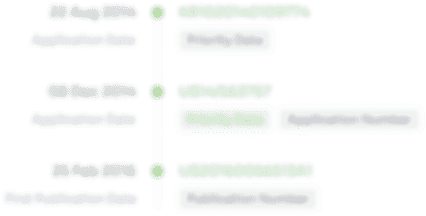
- R&D Engineer
- R&D Manager
- IP Professional
- Industry Leading Data Capabilities
- Powerful AI technology
- Patent DNA Extraction
Browse by: Latest US Patents, China's latest patents, Technical Efficacy Thesaurus, Application Domain, Technology Topic, Popular Technical Reports.
© 2024 PatSnap. All rights reserved.Legal|Privacy policy|Modern Slavery Act Transparency Statement|Sitemap|About US| Contact US: help@patsnap.com