Credit card transaction risk prediction method based on federated learning
A risk prediction and credit card technology, applied in the field of financial data security, can solve problems such as unstable modules, limited improvement, and inapplicability, and achieve the effects of expanding feature dimensions, wide application range, and improving accuracy
- Summary
- Abstract
- Description
- Claims
- Application Information
AI Technical Summary
Problems solved by technology
Method used
Image
Examples
Embodiment Construction
[0047] The present invention will be described in detail below in conjunction with the accompanying drawings and specific embodiments. This embodiment is carried out on the premise of the technical solution of the present invention, and detailed implementation and specific operation process are given, but the protection scope of the present invention is not limited to the following embodiments.
[0048] When China's land, labor, and capital drive economic growth through factorization, the next growth point is data. However, the premise of using data is to break the data island, which explains why the promotion of data integration is crucial. Because, in the process of improving the ability of artificial intelligence and machine learning to implement financial business, data is the only main axis in this upgrading process. Artificial intelligence is developing rapidly, but some of the daily life around us is small data. For example, there are a lot of data in finance, which is ...
PUM
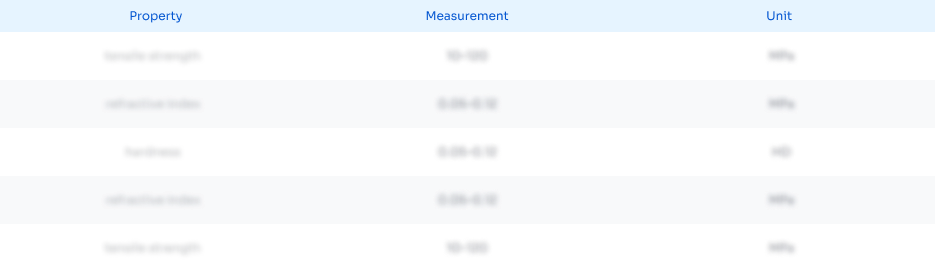
Abstract
Description
Claims
Application Information
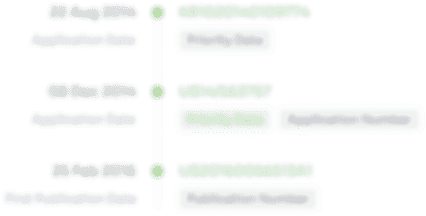
- R&D Engineer
- R&D Manager
- IP Professional
- Industry Leading Data Capabilities
- Powerful AI technology
- Patent DNA Extraction
Browse by: Latest US Patents, China's latest patents, Technical Efficacy Thesaurus, Application Domain, Technology Topic, Popular Technical Reports.
© 2024 PatSnap. All rights reserved.Legal|Privacy policy|Modern Slavery Act Transparency Statement|Sitemap|About US| Contact US: help@patsnap.com