Sign language recognition method and system based on double-flow space-time diagram convolutional neural network
A technology of convolutional neural network and recognition method, applied in the cross field of machine translation, which can solve the problems of weak feature robustness, interference of visual information, and inability to describe information in time domain.
- Summary
- Abstract
- Description
- Claims
- Application Information
AI Technical Summary
Problems solved by technology
Method used
Image
Examples
Embodiment Construction
[0072] The technical scheme of the present invention is described in detail below in conjunction with accompanying drawing:
[0073] like figure 1 As shown, a sign language recognition method based on two-stream spatio-temporal graph convolution disclosed in the present invention uses a bottom-up human body pose estimation method and a hand sign model to detect sign language action videos and extract human skeleton joint point information to construct a human body Skeleton key point map data; use the spatio-temporal graph convolutional neural network to extract the global spatio-temporal feature sequence and local spatio-temporal feature sequence of the video sequence from the upper torso skeleton map data and the hand map data respectively, and perform feature splicing to obtain the global-local spatio-temporal feature sequence Feature sequence; then use the self-attention encoding and decoding network to serialize the spatio-temporal features; finally, obtain the maximum cla...
PUM
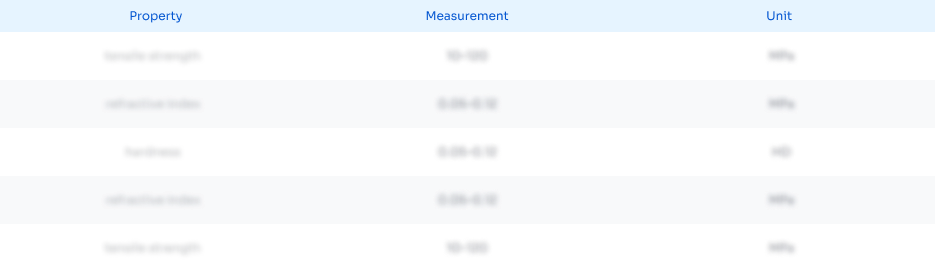
Abstract
Description
Claims
Application Information
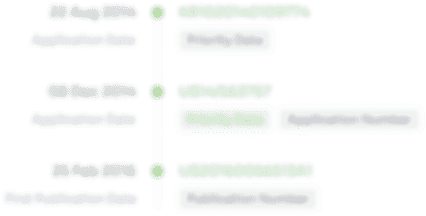
- R&D Engineer
- R&D Manager
- IP Professional
- Industry Leading Data Capabilities
- Powerful AI technology
- Patent DNA Extraction
Browse by: Latest US Patents, China's latest patents, Technical Efficacy Thesaurus, Application Domain, Technology Topic, Popular Technical Reports.
© 2024 PatSnap. All rights reserved.Legal|Privacy policy|Modern Slavery Act Transparency Statement|Sitemap|About US| Contact US: help@patsnap.com