Deep learning-based rail wagon bearing oil shedding fault detection method
A technology for fault detection and railway wagons, applied in neural learning methods, image data processing, biological neural network models, etc., can solve problems completed by artificial naked eyes, achieve reliable fault detection results, high real-time performance, and solve difficult-to-obtain problems Effect
- Summary
- Abstract
- Description
- Claims
- Application Information
AI Technical Summary
Problems solved by technology
Method used
Image
Examples
specific Embodiment approach 1
[0045] A deep learning-based detection method for bearing oil rejection faults of railway wagons, comprising the following steps:
[0046] Step S1: Use a high-speed camera to obtain the whole vehicle image of the railway freight car when it is running. There are multiple parts such as side frame, middle part I, middle part II, coupler and coupler. Use the image naming rules to filter the parts, and only select the parts containing bearings. The image of the side frame part, the size of the image is 1400×1024×3.
[0047] Step S2: Use the Hough circle transform to detect the circle on the image of the above-mentioned side frame, and filter the interfering circle by setting different radius thresholds, so as to locate the bearing area of the side frame, and cut the bearing area to form a fault detection The training set of the network. The radius threshold finally adopted by the present invention is 150-180 pixels.
[0048]Step S3: Based on the research of deep anomaly detect...
specific Embodiment approach 2
[0073] The difference between this embodiment and specific embodiment 1 is that the abnormality discrimination mechanism adopted in step S5 is reconstruction abnormality discrimination mechanism, and the abnormality score is expressed as:
[0074]
[0075] The reconstruction discriminative mechanism represents the difference between the input image and the reconstructed image. First, the input image is reconstructed through the encoder Encoder1 and the decoder Decoder, and the difference between the input image and the reconstructed image is calculated, and then normalized to obtain the abnormal score.
specific Embodiment approach 3
[0076] The difference between this embodiment and specific embodiment 1 is that the abnormality discrimination mechanism adopted in step S5 is a fusion abnormality discrimination mechanism, and the abnormality score is expressed as:
[0077] A t =A r +αA e
PUM
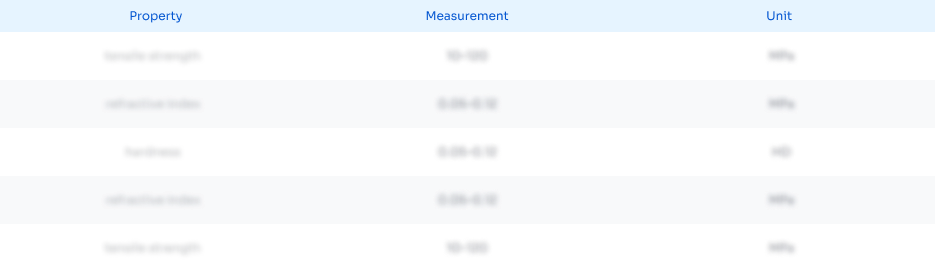
Abstract
Description
Claims
Application Information
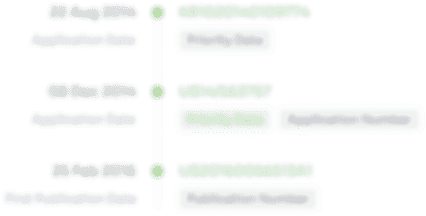
- Generate Ideas
- Intellectual Property
- Life Sciences
- Materials
- Tech Scout
- Unparalleled Data Quality
- Higher Quality Content
- 60% Fewer Hallucinations
Browse by: Latest US Patents, China's latest patents, Technical Efficacy Thesaurus, Application Domain, Technology Topic, Popular Technical Reports.
© 2025 PatSnap. All rights reserved.Legal|Privacy policy|Modern Slavery Act Transparency Statement|Sitemap|About US| Contact US: help@patsnap.com