ST-GRU memristor neural network circuit for realizing gait prediction and training method
A ST-GRU, neural network technology, applied in the field of neural network circuits, can solve problems such as inability to target training, and achieve the effect of strengthening the ability to deal with dynamic prediction problems, high accuracy, and good computing efficiency
- Summary
- Abstract
- Description
- Claims
- Application Information
AI Technical Summary
Problems solved by technology
Method used
Image
Examples
Embodiment approach
[0034] (1) The GRU unit circuit for processing time series is mainly composed of a memristor array; the GRU unit circuit for processing space series is mainly composed of a memristor array; for example: the time series for processing The GRU unit circuit can be employed as Figure 4 The circuit shown ( Figure 4 The memristor array is represented in the three boxes of ), the GRU unit circuit for processing spatial sequences can be adopted as Figure 4 The circuit shown ( Figure 4 The memristor array is represented in three boxes). Because the memristor has a non-volatile memory function, its volume and power consumption are smaller than traditional memory, and it does not need to refresh regularly like DRAM, so the present invention preferably selects the memristor as the unit of the neural network structure.
[0035] (2) The GRU unit circuit for processing the time series processes the time characteristic pulse voltage signal at the current time in the time series as the ...
Embodiment 1
[0047] Such as figure 2 , image 3 and Figure 4 As shown, a ST-GRU memristive neural network circuit for gait prediction, its structure includes: GRU unit circuit for processing time series, GRU unit circuit and fully connected layer circuit for processing space series; time series Each moment in each corresponds to a space sequence; the GRU unit circuit for processing the time sequence processes the time characteristic pulse voltage signal at each moment in the time sequence, and the GRU unit circuit for processing the space sequence The characteristic pulse voltage signal of the spatial sequence corresponding to each moment is processed.
[0048] For the time characteristic pulse voltage signal at each moment in the time series, after the GRU unit circuit for processing the time series processes the time characteristic pulse voltage signal at a moment in the time series, the The output result of the GRU unit circuit is used as the spatial characteristic pulse voltage si...
PUM
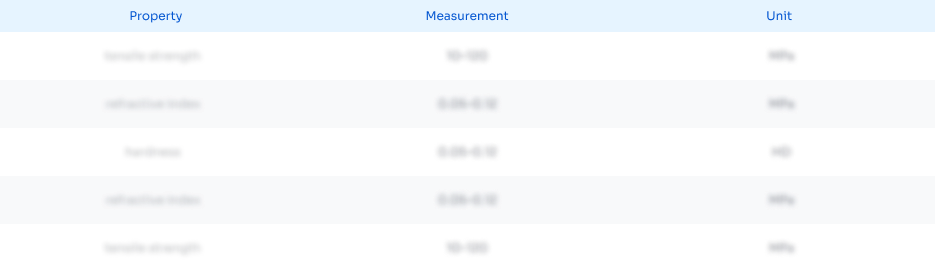
Abstract
Description
Claims
Application Information
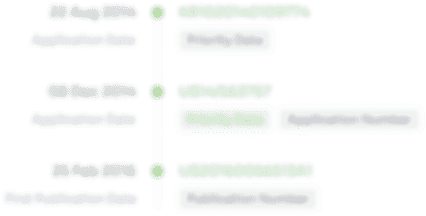
- R&D Engineer
- R&D Manager
- IP Professional
- Industry Leading Data Capabilities
- Powerful AI technology
- Patent DNA Extraction
Browse by: Latest US Patents, China's latest patents, Technical Efficacy Thesaurus, Application Domain, Technology Topic, Popular Technical Reports.
© 2024 PatSnap. All rights reserved.Legal|Privacy policy|Modern Slavery Act Transparency Statement|Sitemap|About US| Contact US: help@patsnap.com