Real-time monthly runoff forecasting method based on deep learning model
A deep learning and runoff technology, applied in neural learning methods, biological neural network models, forecasting, etc., can solve problems such as large arbitrariness and uncertainty, single source of predictors, monthly runoff forecast gap, etc., to maintain forecasting accuracy , the effect of good forecast accuracy
- Summary
- Abstract
- Description
- Claims
- Application Information
AI Technical Summary
Problems solved by technology
Method used
Image
Examples
Embodiment Construction
[0047] The real-time monthly runoff forecasting method based on the deep learning model involved in the present invention will be described in detail below in conjunction with the accompanying drawings.
[0048]
[0049] Such as figure 1 As shown, the real-time monthly runoff forecasting method based on the deep learning model provided in this embodiment includes the following steps:
[0050] Step 1. Collect forecast factors based on historical information and future meteorological information, and determine the longest lag time of the influence of previous monthly runoff on the forecast month according to the autocorrelation analysis of monthly runoff in the watershed's historical period, and use this value as the value of other predictors Maximum impact lag. Step 1 further includes the following sub-steps:
[0051] Step 1.1 The candidate predictors collected in this embodiment include historical information and future information. All the following candidate predictors a...
PUM
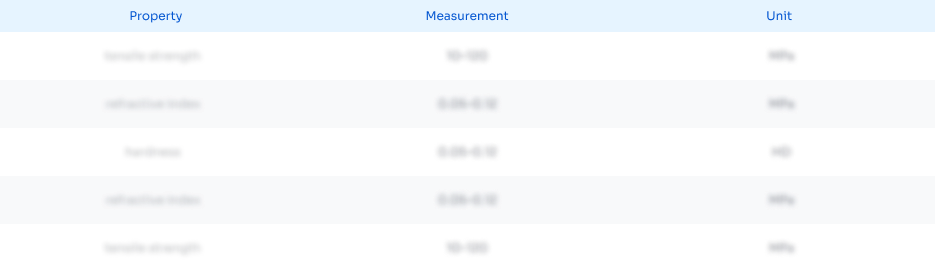
Abstract
Description
Claims
Application Information
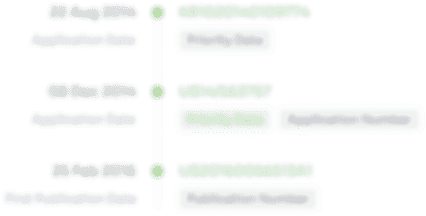
- R&D Engineer
- R&D Manager
- IP Professional
- Industry Leading Data Capabilities
- Powerful AI technology
- Patent DNA Extraction
Browse by: Latest US Patents, China's latest patents, Technical Efficacy Thesaurus, Application Domain, Technology Topic.
© 2024 PatSnap. All rights reserved.Legal|Privacy policy|Modern Slavery Act Transparency Statement|Sitemap