Optimization method for steganalysis of convolutional neural network
A convolutional neural network and steganalysis technology, applied in the field of spatial domain image information hiding and steganalysis, can solve the problem of heavy workload, increase the computational complexity of feature classification, workload, work complexity and work difficulty, etc. problem, to achieve the effect of improving the accuracy
- Summary
- Abstract
- Description
- Claims
- Application Information
AI Technical Summary
Problems solved by technology
Method used
Image
Examples
Embodiment Construction
[0046] In order to make the above-mentioned features and advantages of the present invention more comprehensible, the present invention will be further described in detail below in conjunction with specific embodiments and accompanying drawings. The specific CNN steganalysis training process is as follows: figure 1 As shown, its main steps include:
[0047] Step 101, adding a high-pass filter to the shallowest layer of the model to obtain a noise residual image.
[0048] Step 102, performing dimensionality reduction processing on the sample points, so that observation can be performed in a two-dimensional visual situation ( figure 2 ).
[0049] Step 103, constructing the characteristic variation coefficients of the two types of samples. Feature Learning Capabilities for Comparing Multiple Convolutional Neural Network Steganographic Detection Algorithms
[0050] Step 103, calculate coefficient of variation and modify feature ( Figure 4 ), the improved CNN model is obtain...
PUM
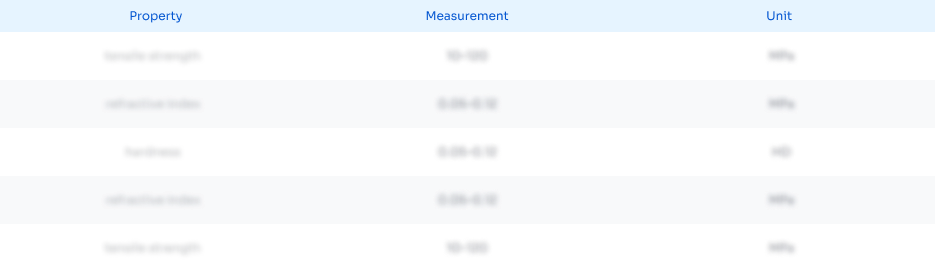
Abstract
Description
Claims
Application Information
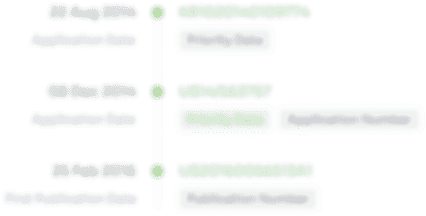
- R&D Engineer
- R&D Manager
- IP Professional
- Industry Leading Data Capabilities
- Powerful AI technology
- Patent DNA Extraction
Browse by: Latest US Patents, China's latest patents, Technical Efficacy Thesaurus, Application Domain, Technology Topic, Popular Technical Reports.
© 2024 PatSnap. All rights reserved.Legal|Privacy policy|Modern Slavery Act Transparency Statement|Sitemap|About US| Contact US: help@patsnap.com