Multi-sensor fusion convolutional neural network aero-engine bearing fault diagnosis method
A convolutional neural network, multi-sensor fusion technology, applied in neural learning methods, biological neural network models, neural architectures, etc., can solve problems such as difficult to accurately identify, unclear fault characteristics, etc., to achieve the effect of information processing
- Summary
- Abstract
- Description
- Claims
- Application Information
AI Technical Summary
Problems solved by technology
Method used
Image
Examples
Embodiment 1
[0043] Embodiment 1 of the present application provides a multi-sensor fusion convolutional neural network aeroengine bearing fault diagnosis method, including: data collection, data preprocessing, data storage, source domain 1D-CNN model offline training, target domain bearing online diagnosis, wherein :
[0044] Data acquisition part: Arrange vibration acceleration sensors according to actual needs, and collect acceleration signals at different positions and directions of the equipment.
[0045]Data preprocessing part: It is necessary to normalize, slice and label the collected original state parameters, and convert them into data types that can be recognized by 1D-CNN.
[0046] Further, the normalization process adopts maximum and minimum value normalization, and the formula is:
[0047]
[0048] Where: x max is the maximum value of the sample data, x min is the minimum value of the sample data, x` is the normalized result, and the value interval is [0, 1].
[0049] ...
Embodiment 2
[0071] Such as figure 2 Shown is a multi-sensor fusion convolutional neural network aero-engine bearing fault diagnosis method flow chart, including: data acquisition and preprocessing, offline training, online diagnosis. Specifically include the following steps:
[0072] S1. Data collection: Collect the relevant data of deep groove ball bearings tested on a helicopter transmission system test bench and the main reduction test bench. The sampling frequency is 10,000 Hz, and the sampling time is 3 minutes, that is, 1,800,000 data points are sampled for each fault type; Bearing fault types are outer ring fault, inner ring fault, rolling element fault, combined fault and normal bearing;
[0073] S2. Data preprocessing: normalize, slice, and label the data, and convert the data into a data type that can be used for supervised learning. The data structure is shown in Table 1:
[0074] Table 1 Labels of the gear dataset
[0075] Number of samples (training set / test set...
Embodiment 3
[0081] According to the 1D-CNN neural network model and the principle of multi-sensor information fusion, the specific structural parameters of the aeroengine bearing fault diagnosis model proposed by the present invention are shown in Table 2.
[0082] The 1D-CNN established by the present invention for aeroengine bearing fault diagnosis is composed of four sub-models with the same parameters, and each sub-model includes four sets of convolution-maximum pooling layers and one average pooling layer. The output of the last layer of each sub-model is aggregated and input to the same Flatten layer for flattening, and finally the recognition result is output in the Softmax layer. After the original bearing data passes through the convolutional layer, it is converted into a set of feature maps (multi-channel one-dimensional array), and then it is down-sampled by maximum pooling, thereby reducing the number of parameters. After these operations are repeated 3 times, the characterist...
PUM
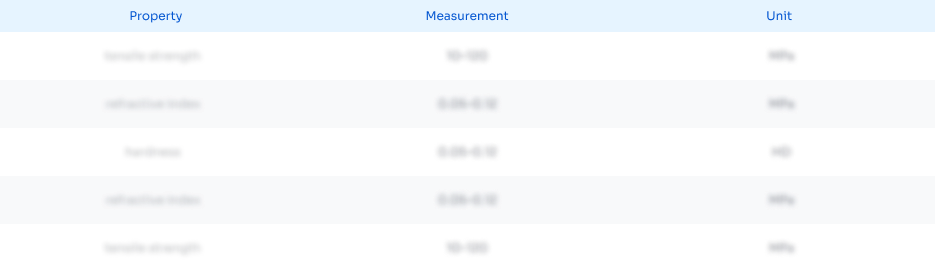
Abstract
Description
Claims
Application Information
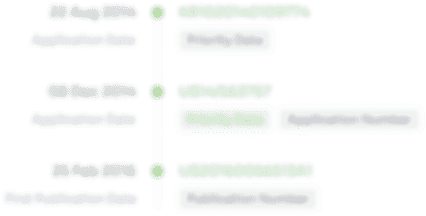
- R&D Engineer
- R&D Manager
- IP Professional
- Industry Leading Data Capabilities
- Powerful AI technology
- Patent DNA Extraction
Browse by: Latest US Patents, China's latest patents, Technical Efficacy Thesaurus, Application Domain, Technology Topic, Popular Technical Reports.
© 2024 PatSnap. All rights reserved.Legal|Privacy policy|Modern Slavery Act Transparency Statement|Sitemap|About US| Contact US: help@patsnap.com