Air pollutant concentration prediction method based on graph attention mechanism
A technology for predicting air pollutants and concentrations, applied in prediction, neural learning methods, measuring devices, etc., can solve problems such as high data quality requirements, large data volume, and long time consumption
- Summary
- Abstract
- Description
- Claims
- Application Information
AI Technical Summary
Problems solved by technology
Method used
Image
Examples
Embodiment Construction
[0064] The present invention will be described in further detail through examples below in conjunction with the accompanying drawings, but the scope of the present invention is not limited in any way.
[0065] A spatial pollutant concentration prediction algorithm based on a graph attention mechanism proposed by the present invention combines meteorological station monitoring data, air monitoring data, and environmental factor data as model input data, constructs a graph adjacency matrix through a graph attention mechanism, and combines graph The convolutional neural network layer and the multi-layer perceptron network layer extract the image information features, and finally output the predicted air pollutant concentration value. The overall implementation process of the method is as follows figure 1 As shown, it includes two processes of training phase and testing phase.
[0066] A method for predicting the concentration of spatial pollutants based on a graph attention mech...
PUM
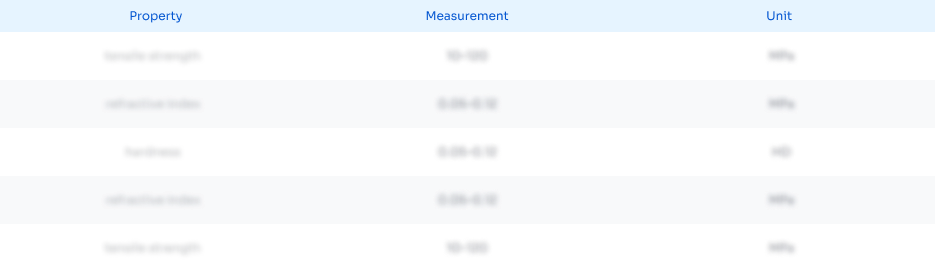
Abstract
Description
Claims
Application Information
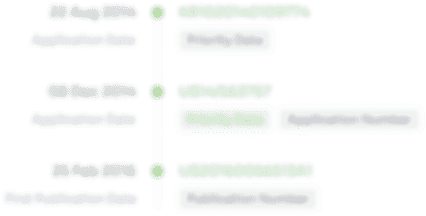
- R&D Engineer
- R&D Manager
- IP Professional
- Industry Leading Data Capabilities
- Powerful AI technology
- Patent DNA Extraction
Browse by: Latest US Patents, China's latest patents, Technical Efficacy Thesaurus, Application Domain, Technology Topic, Popular Technical Reports.
© 2024 PatSnap. All rights reserved.Legal|Privacy policy|Modern Slavery Act Transparency Statement|Sitemap|About US| Contact US: help@patsnap.com