A Convolutional Neural Network Object Detection Method Based on Pyramid Input Gain
A convolutional neural network and target detection technology, which is applied in biological neural network models, neural architecture, image enhancement, etc., can solve the problems of high missed detection rate and low reliability, and achieve accuracy assurance, wide application, and solution The effect of precision loss
- Summary
- Abstract
- Description
- Claims
- Application Information
AI Technical Summary
Problems solved by technology
Method used
Image
Examples
Embodiment
[0051] According to the method steps described in the summary of the invention, a PiaNet network model structure corresponding to an embodiment of the present invention for detecting pulmonary nodules on CT images is as follows figure 1 shown.
[0052] Step (1) data preprocessing;
[0053] The original image is preprocessed by de-meaning and grayscale normalization to obtain the preprocessed image, where the original CT input image is as figure 2 shown;
[0054] Step (2) inputs the preprocessed image output of step (1) into the PiaNet network;
[0055] Step (2) comprises the following sub-steps again:
[0056] Step (2A) The input image is subjected to an average pooling operation on the source connection path to obtain a compressed source image. Among them, the multi-scale source image generated by multi-level average pooling can form an image pyramid, such as image 3 shown;
[0057] Step (2B) At the same time, the input image undergoes feature extraction through convo...
PUM
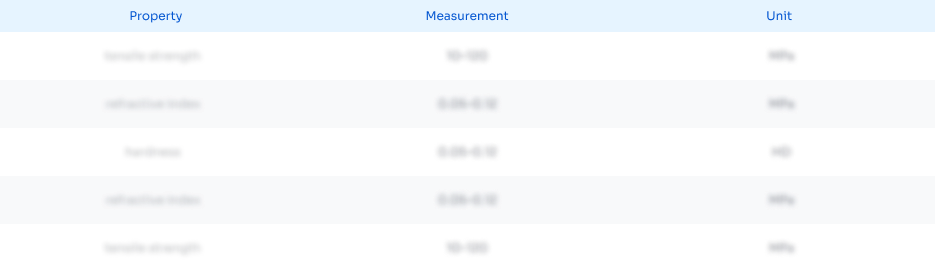
Abstract
Description
Claims
Application Information
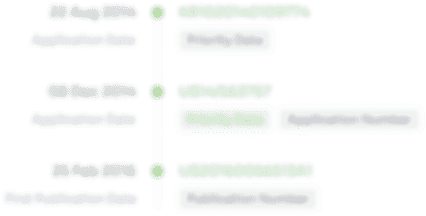
- R&D Engineer
- R&D Manager
- IP Professional
- Industry Leading Data Capabilities
- Powerful AI technology
- Patent DNA Extraction
Browse by: Latest US Patents, China's latest patents, Technical Efficacy Thesaurus, Application Domain, Technology Topic, Popular Technical Reports.
© 2024 PatSnap. All rights reserved.Legal|Privacy policy|Modern Slavery Act Transparency Statement|Sitemap|About US| Contact US: help@patsnap.com