Brain tumor image segmentation algorithm based on improved full-convolution neural network
A convolutional neural network and image segmentation technology, applied in the field of medical imaging, can solve the problem of low segmentation accuracy of brain tumor images and achieve strong stability
- Summary
- Abstract
- Description
- Claims
- Application Information
AI Technical Summary
Problems solved by technology
Method used
Image
Examples
Embodiment Construction
[0031] The brain tumor image segmentation algorithm process of the improved fully convolutional neural network of the present invention is as follows: figure 1shown. Firstly, grayscale image fusion is performed on the normalized multimodal MR brain tumor image data; then part of the fused data is used as a training set, and part of the data is used as a test set, and the training set is used to train the fusion algorithm of FCNN and CRF; finally, in the test The test of the model and the index evaluation of the segmentation results are carried out on the set.
[0032] 1) Image preprocessing
[0033] Each pixel of the original MR brain tumor image is 16bit, but in the digital image processing technology, the 8bit image is processed, so the present invention first normalizes the grayscale of the MR image, and the grayscale of each pixel is Degree values are uniformly compressed to a range between 0-255. Considering that the features extracted by the neural network only thro...
PUM
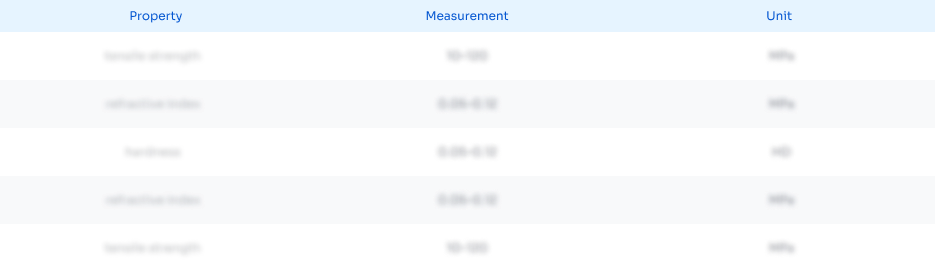
Abstract
Description
Claims
Application Information
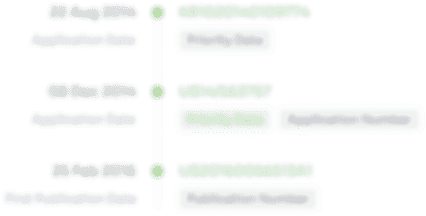
- R&D Engineer
- R&D Manager
- IP Professional
- Industry Leading Data Capabilities
- Powerful AI technology
- Patent DNA Extraction
Browse by: Latest US Patents, China's latest patents, Technical Efficacy Thesaurus, Application Domain, Technology Topic, Popular Technical Reports.
© 2024 PatSnap. All rights reserved.Legal|Privacy policy|Modern Slavery Act Transparency Statement|Sitemap|About US| Contact US: help@patsnap.com