Electroencephalogram signal classification method based on model uncertainty learning
An EEG signal and uncertainty technology, applied in neural learning methods, biological neural network models, character and pattern recognition, etc., can solve the problems of insufficient training data and high cost of data collection, and achieve the effect of enhancing reliability.
- Summary
- Abstract
- Description
- Claims
- Application Information
AI Technical Summary
Problems solved by technology
Method used
Image
Examples
Embodiment Construction
[0033]In this embodiment, an EEG signal classification method based on model uncertainty learning mainly uses a lightweight network (PeleeNet) to achieve a balance between high performance and few model parameters, and the improved Monte Carlo discarding technology uses short There is a great similarity between EEG samples in time. Combined with the time information of continuous samples, the calibrated classification model is obtained by predicting and averaging consecutive samples through the dropout layer, such as figure 1 Specifically, the method is as follows:
[0034] Step 1. Obtain an EEG data set with labeled category information, and select channel data for the original EEG signals in the EEG data set to obtain C channels of EEG signals, and then pass the sliding window (such as figure 2 shown) slice the EEG signals of C channels, and reconstruct the input shape of the sliced EEG signals, so as to obtain N-segment EEG samples with a total duration of T, denoted as ...
PUM
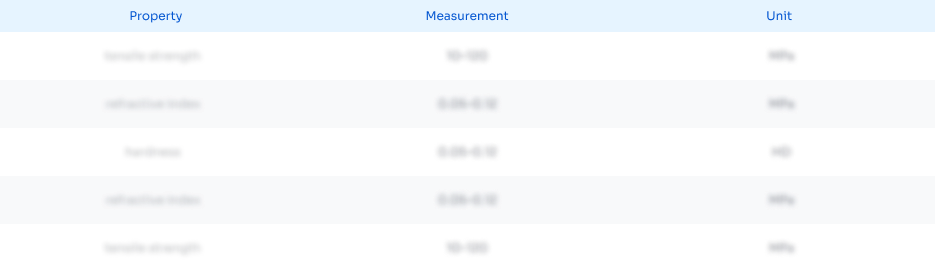
Abstract
Description
Claims
Application Information
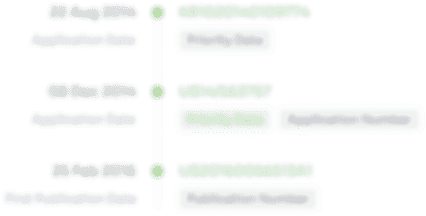
- R&D Engineer
- R&D Manager
- IP Professional
- Industry Leading Data Capabilities
- Powerful AI technology
- Patent DNA Extraction
Browse by: Latest US Patents, China's latest patents, Technical Efficacy Thesaurus, Application Domain, Technology Topic, Popular Technical Reports.
© 2024 PatSnap. All rights reserved.Legal|Privacy policy|Modern Slavery Act Transparency Statement|Sitemap|About US| Contact US: help@patsnap.com