Method for accelerating multi-outlet DNN reasoning by heterogeneous processor under edge computing
A heterogeneous processor and edge computing technology, applied in neural learning methods, neural architecture, biological neural network models, etc., can solve problems such as inability to meet edge intelligent applications, low latency, etc., to improve user experience and ensure stability. Effect
- Summary
- Abstract
- Description
- Claims
- Application Information
AI Technical Summary
Problems solved by technology
Method used
Image
Examples
Embodiment 1
[0066] Example 1: as figure 1 As shown, the present invention predicts the computation time overhead required by each network layer by training a delay prediction model, and uses this to represent the computation amount of each network layer. When only model parameters are considered, the amount of computation of each layer is mainly represented by floating-point operations (FLOPs). The types of layers include convolutional layers, pooling layers, excitation layers and fully connected layers. Important parameters that determine the amount of computation include: The size of the input feature map (W×H), the number of input and output channels (C in , C out ), the convolution kernel window size (k w ×k h ); pooling window (p w ×p h ), and the number of input and output neurons of the fully connected layer (F in , F out ), the floating-point operation is calculated as follows:
[0067]
[0068] Combined with the actual system, considering the influence of CPU usage (u)...
Embodiment 2
[0071] Example 2: as figure 1 As shown, under different CPU utilization conditions, there are different execution strategies corresponding to different execution times. Based on the time delay quantification prediction model obtained by the above-mentioned multi-path model, the "early exit probability" of the branch and the "data amount transmission delay of the intermediate feature", the multi-exit deep neural network is segmented by the segmentation prediction model. The invention parallelizes the inference process of the model backbone and the egress network, thereby improving resource utilization while reducing the inference delay of multi-path, and solving the problem of performance drop caused by redundant computing in multi-egress network inference in extreme cases . In the model segmentation strategy, it is necessary to specify the model segmentation decision variables Among them, 1-n represents n branches, the main part is executed by the GPU, and the network model...
Embodiment 3
[0077] Embodiment 3: Asynchronous execution of multi-exit network inference work. like figure 2 As shown, the online stage includes model segmentation and cooperative multi-path parallel inference steps of heterogeneous processors. After all offline stages are realized, the generated multi-path deep neural network is deployed on the heterogeneous processors of mobile smart terminal devices. , which performs online multipath parallel inference. The intelligent terminal device monitors the local computing load in real time, and predicts the corresponding task allocation decision X according to the real-time load situation after receiving the task. b , the execution of the task is divided into two parallel parts: the inference of the trunk network and the inference of the branch-exit network. When the branch-exit network is assigned to the CPU for execution, if the task can exit from the branch-exit network, the execution of the trunk network will stop further. Hierarchical re...
PUM
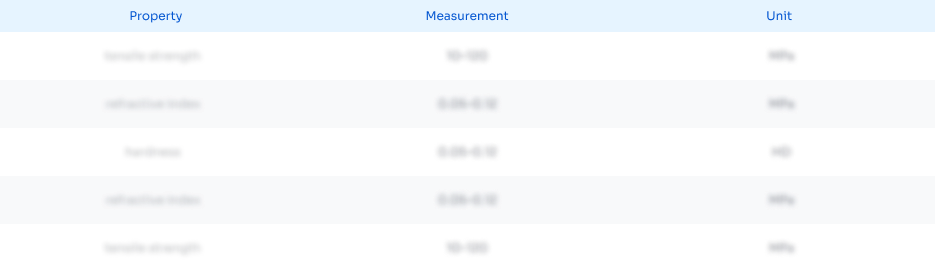
Abstract
Description
Claims
Application Information
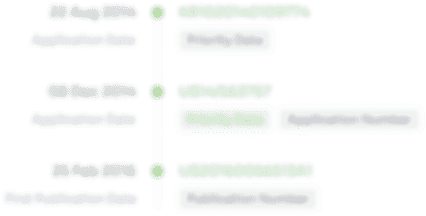
- Generate Ideas
- Intellectual Property
- Life Sciences
- Materials
- Tech Scout
- Unparalleled Data Quality
- Higher Quality Content
- 60% Fewer Hallucinations
Browse by: Latest US Patents, China's latest patents, Technical Efficacy Thesaurus, Application Domain, Technology Topic, Popular Technical Reports.
© 2025 PatSnap. All rights reserved.Legal|Privacy policy|Modern Slavery Act Transparency Statement|Sitemap|About US| Contact US: help@patsnap.com