Federal learning privacy protection system and method based on hierarchical aggregation and block chain
A privacy protection system and hierarchical aggregation technology, applied in the fields of digital data protection, instruments, computing, etc., can solve the problems of low efficiency of federated learning privacy protection mechanism, reduced model accuracy, large storage overhead, etc., and achieve traceability and model integrity. Sexual security, improve efficiency, and ensure the effect of integrity
- Summary
- Abstract
- Description
- Claims
- Application Information
AI Technical Summary
Problems solved by technology
Method used
Image
Examples
Embodiment Construction
[0028] Definition and Explanation of Terms:
[0029] Federated learning: Federated learning is a machine learning technique that specifically trains algorithms on multiple distributed edge devices or servers with local data samples. This approach differs significantly from traditional centralized machine learning techniques that upload all local datasets to a single server. Federated learning enables multiple participants to jointly train a machine learning model by sharing local model parameters without sharing data, thereby solving key issues such as data privacy, data security, and data access rights.
[0030] Hierarchical aggregation: Hierarchical aggregation means that in the federated learning parameter aggregation process, the user does not directly send the parameters to the aggregator, but divides the parameters and distributes them to each sub-aggregator, and each sub-aggregator initially aggregates the parameters and then Sent to the aggregator to complete a round ...
PUM
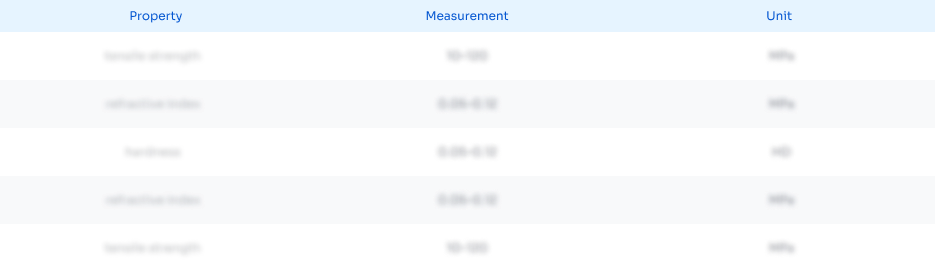
Abstract
Description
Claims
Application Information
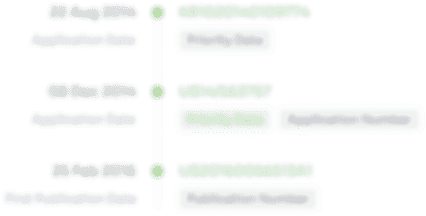
- R&D Engineer
- R&D Manager
- IP Professional
- Industry Leading Data Capabilities
- Powerful AI technology
- Patent DNA Extraction
Browse by: Latest US Patents, China's latest patents, Technical Efficacy Thesaurus, Application Domain, Technology Topic, Popular Technical Reports.
© 2024 PatSnap. All rights reserved.Legal|Privacy policy|Modern Slavery Act Transparency Statement|Sitemap|About US| Contact US: help@patsnap.com