Acute myelogenous leukemia drug sensitivity related gene classifier constructed by machine learning algorithm
An acute myeloid and machine learning technology, applied in the field of leukemia research, can solve the problems of lack of consistency of drug resistance and other problems
- Summary
- Abstract
- Description
- Claims
- Application Information
AI Technical Summary
Problems solved by technology
Method used
Image
Examples
Embodiment 1
[0102] A machine learning algorithm constructs a drug-sensitivity-related gene classifier for acute myeloid leukemia, and the specific algorithm is:
[0103] Cluster analysis of drugs
[0104] The K-means clustering algorithm was used to group the patients' drug sensitivity (K=2), so that the patients were divided into two groups, and a data set with classification labels was provided for the subsequent screening of drug-sensitive genes using the supervised learning algorithm.
[0105] The specific steps of the K-Means algorithm
[0106] (1) Randomly select 2 samples C from the processed samples 1 , C 2 as the initial cluster center.
[0107] (2) According to the data of each sample, calculate the distance between each sample and two cluster centers, and divide it into the class corresponding to the cluster center with the smallest distance.
[0108] The distance measure between the sample and the cluster center is Euclidean distance:
[0109] Among them, x represents t...
Embodiment 2
[0158] In this example, a total of 41 patients with relapsed and refractory AML and newly diagnosed AML were included, and transcriptome RNA-seq sequencing and methylomics 850K chip sequencing were performed at the same time. A total of 598,243 methylated gene probe sites are involved, and a total of 23,710 genes are involved in the transcriptome. Considering the limited number of samples and the large number of sample feature genes, all gene feature data are used for modeling, which is prone to failure of high-dimensional features. As a result, the accuracy of model learning is lost. Therefore, this paper first considers the differential analysis of gene features, and then performs dimensionality reduction in different algorithm modes.
[0159] ChAMP method was used for differential analysis of methylomics and DESeq2 method for differential analysis of transcriptome data. Then, based on the difference analysis, the feature dimensionality reduction of the original data is car...
Embodiment 3
[0162] GDSC database verification screening gene drug sensitivity prediction accuracy
[0163] IC50 (half inhibitory concentration) is the main evaluation index for the therapeutic effect of GDSC database drugs in cell lines. In this study, the R package pRRophetic version 0.5 was used to obtain and integrate the GDSC database. The pRRophetic package was developed by Paul Geeleher in 2014. It selected the clinical response of 138 drugs from more than 700 cell lines included in the Cancer Genome Project (CGP) database, and developed a drug response prediction algorithm using the expression matrix of the CGP database. The reliability of the algorithm is verified in the data set. The basic principles and steps are as follows:
[0164] 1) Standardize the CGP database (cell line gene expression matrix as a training set) and the expression matrix to be predicted (clinical patient gene expression matrix as a test set) respectively, and select empirical Bayesian method to merge data ...
PUM
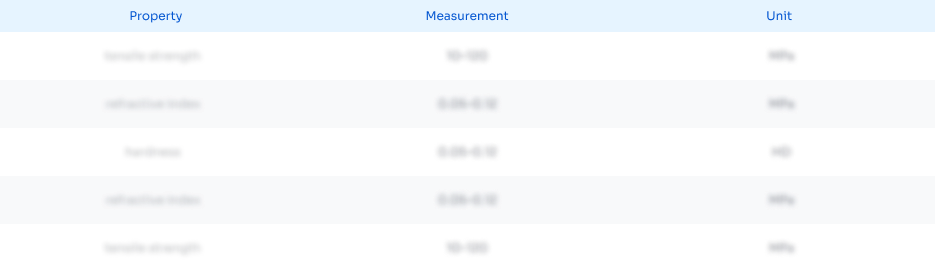
Abstract
Description
Claims
Application Information
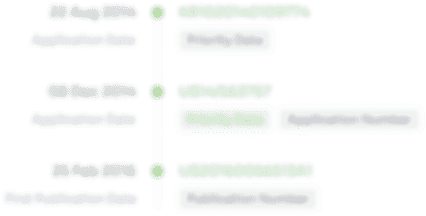
- R&D Engineer
- R&D Manager
- IP Professional
- Industry Leading Data Capabilities
- Powerful AI technology
- Patent DNA Extraction
Browse by: Latest US Patents, China's latest patents, Technical Efficacy Thesaurus, Application Domain, Technology Topic, Popular Technical Reports.
© 2024 PatSnap. All rights reserved.Legal|Privacy policy|Modern Slavery Act Transparency Statement|Sitemap|About US| Contact US: help@patsnap.com