An Interpretable Deep Neural Network Fault Diagnosis Method for Air Conditioning Systems
A deep neural network and fault diagnosis technology, applied in neural learning methods, biological neural network models, neural architectures, etc., can solve problems such as poor interpretability, and achieve the effect of high accuracy and enhanced interpretability
- Summary
- Abstract
- Description
- Claims
- Application Information
AI Technical Summary
Problems solved by technology
Method used
Image
Examples
Embodiment
[0038] Embodiment: An interpretable deep neural network fault diagnosis method for air-conditioning systems, based on a deep learning model, using a one-dimensional convolutional neural network model to extract feature information of HVAC operating data, and using the absolute gradient weighted class activation map as a visualization Classification diagnostic criteria, using the absolute gradient weighted class activation map to visualize the fault data information of the HVAC system, and obtaining the fault diagnosis criteria corresponding to each fault data in the one-dimensional convolutional neural network model through the absolute gradient weighted class activation map, so that the fault diagnosis model becomes interpretable, as in figure 1 As shown, it specifically includes the following steps:
[0039] S1. Feature learning and fault diagnosis of one-dimensional convolutional neural network model, the specific method is:
PUM
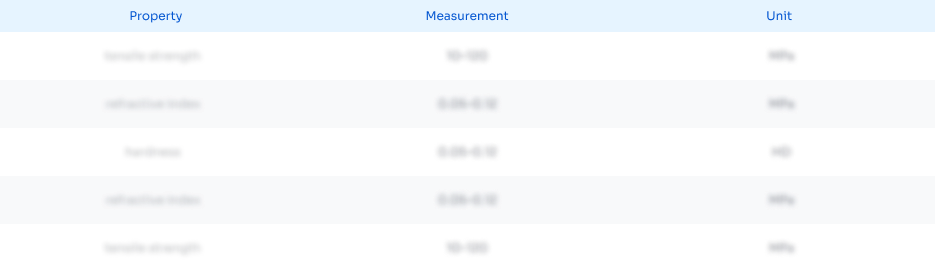
Abstract
Description
Claims
Application Information
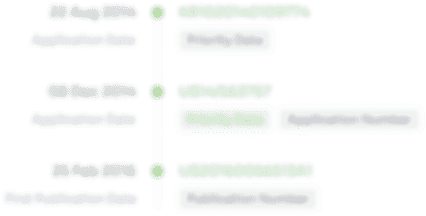
- R&D Engineer
- R&D Manager
- IP Professional
- Industry Leading Data Capabilities
- Powerful AI technology
- Patent DNA Extraction
Browse by: Latest US Patents, China's latest patents, Technical Efficacy Thesaurus, Application Domain, Technology Topic, Popular Technical Reports.
© 2024 PatSnap. All rights reserved.Legal|Privacy policy|Modern Slavery Act Transparency Statement|Sitemap|About US| Contact US: help@patsnap.com