Safety helmet identification method and device based on YOLOv3, medium and equipment
A recognition method and helmet technology, which is applied in the field of image recognition, can solve the problems that the recognition algorithm cannot meet the actual needs of helmet recognition, and achieve the effects of meeting real-time detection requirements, improving safety assurance, improving accuracy and detection speed
- Summary
- Abstract
- Description
- Claims
- Application Information
AI Technical Summary
Problems solved by technology
Method used
Image
Examples
Embodiment 1
[0043] Such as figure 1 Shown:
[0044] The disclosure provides a YOLOv3-based helmet identification method, including:
[0045] S101: collecting construction site image data;
[0046] S102: Use an image annotation tool to annotate the image data to obtain annotated image data;
[0047] S103: Divide the labeled image data set into two parts, a training set and a test set, and divide the image data of the training set into different groups according to different resolutions;
[0048] S104: Utilize the neural network based on the Darknet-53 network model for model training, and obtain a trained helmet recognition model;
[0049] S105: Test the recognition accuracy of the helmet recognition model by using the test set image data through the YOLOv3 algorithm.
[0050] Further, the image annotation tool is a deep learning image annotation labelme tool.
[0051] LabelMe is a Javascript labeling tool for online image labeling. The advantage over traditional image annotation too...
Embodiment 2
[0094] Such as figure 2 as shown,
[0095] The present disclosure can also provide a helmet recognition device based on YOLOv3, including:
[0096] An image acquisition module 201, configured to acquire target image data;
[0097] An image labeling module 202, configured to use an image labeling tool to label the image data to obtain labeled image data;
[0098] An image grouping module 203, configured to divide the marked image data set into two parts, a training set and a test set, and divide the image data of the training set into different groups according to different resolutions;
[0099]The model training module 204 is used to select no less than one group of image data from the different groups of image data, and use the neural network based on Darknet-53 to perform model training on the image data of the training set , get the helmet recognition model after training;
[0100] A model testing module 205, configured to use the test set image data to test the recogn...
Embodiment 3
[0117] The present disclosure can also provide a computer storage medium on which a computer program is stored, and when the computer program is executed by a processor, it is used to realize the steps of the above-mentioned YOLOv3-based helmet identification method.
[0118] The computer storage medium of the present disclosure may be realized by using a semiconductor memory or a magnetic core memory.
[0119] Semiconductor memory, mainly used in computers, mainly has two types of semiconductor memory elements: Mos and bipolar. Mos components are highly integrated, the process is simple but the speed is slow. Bipolar components are complex in process, high in power consumption, low in integration but fast in speed. After the advent of NMos and CMos, Mos memory began to play a major role in semiconductor memory. NMos is fast, for example, the access time of Intel's 1K-bit SRAM is 45ns. CMos consumes less power, and the 4K-bit CMos static memory access time is 300ns. The ab...
PUM
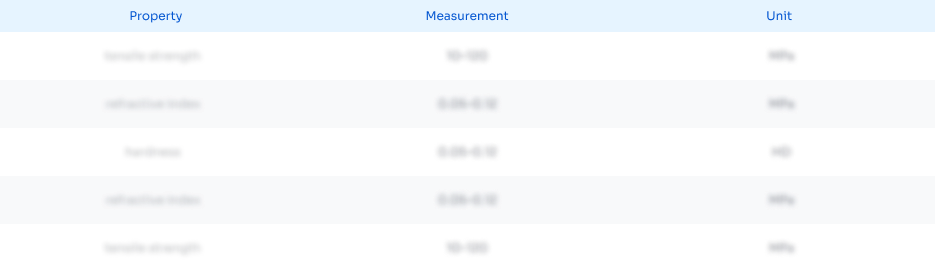
Abstract
Description
Claims
Application Information
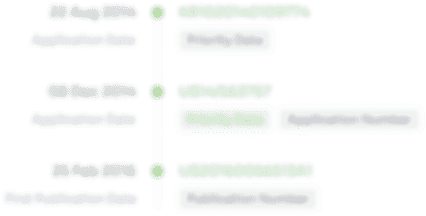
- R&D Engineer
- R&D Manager
- IP Professional
- Industry Leading Data Capabilities
- Powerful AI technology
- Patent DNA Extraction
Browse by: Latest US Patents, China's latest patents, Technical Efficacy Thesaurus, Application Domain, Technology Topic, Popular Technical Reports.
© 2024 PatSnap. All rights reserved.Legal|Privacy policy|Modern Slavery Act Transparency Statement|Sitemap|About US| Contact US: help@patsnap.com