Method for supervising and predicting abnormal furnace condition of blast furnace based on tuyere information deep learning
A technology of deep learning and blast furnace conditions, applied in the field of metallurgy, can solve problems such as blindness, excessive subjectivity, and roughness of manual experience operations
- Summary
- Abstract
- Description
- Claims
- Application Information
AI Technical Summary
Problems solved by technology
Method used
Image
Examples
Embodiment
[0057] The present invention provides a method for supervising and predicting abnormal blast furnace conditions based on deep learning of tuyere information. The process is as follows figure 1 As shown, it specifically includes the following steps:
[0058] Step S1: Collect the video of the blast furnace tuyere swirl area. The original video is continuously extracted at a frequency of 10 minutes per frame. After preprocessing, it is stored in the sample database to form the original tuyere image data set; the preprocessing includes:
[0059] S1A: Aiming at the problem of image blur and noise, the noise type of the image is judged by analyzing the spectrum information and histogram information of the image, and the image of the blast furnace gyration is denoised by using the combination of adaptive median filter and gamma transform. Such as figure 2 shown. The adaptive median filter can not only filter out the salt and pepper noise with high probability, but also better prot...
PUM
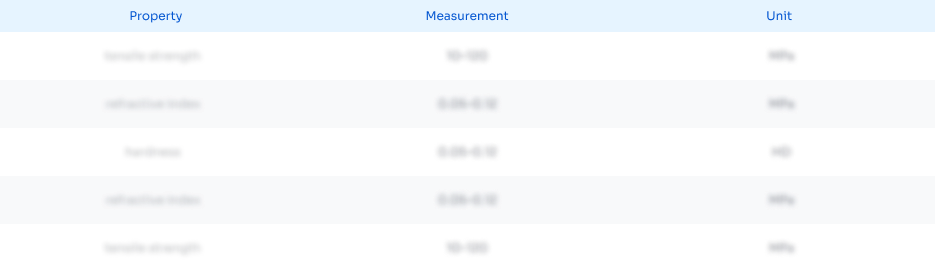
Abstract
Description
Claims
Application Information
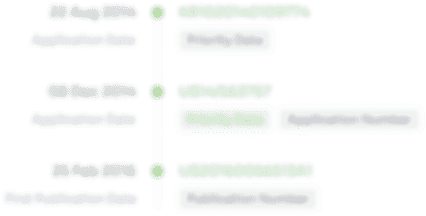
- R&D Engineer
- R&D Manager
- IP Professional
- Industry Leading Data Capabilities
- Powerful AI technology
- Patent DNA Extraction
Browse by: Latest US Patents, China's latest patents, Technical Efficacy Thesaurus, Application Domain, Technology Topic.
© 2024 PatSnap. All rights reserved.Legal|Privacy policy|Modern Slavery Act Transparency Statement|Sitemap