Consumption credit scene fraud detection method based on ABC-SOM neural network
An ABC-SOM, neural network technology, applied in the field of fraud detection, can solve problems such as increasing the computational complexity of the algorithm, slow convergence, and real-time detection of unfavorable consumer credit scenarios.
- Summary
- Abstract
- Description
- Claims
- Application Information
AI Technical Summary
Problems solved by technology
Method used
Image
Examples
Embodiment 1
[0101] see figure 1 , the present invention provides a technical solution:
[0102] A method for detecting fraud in a consumer credit scene based on an ABC-SOM neural network, comprising steps:
[0103] S1: Collect data, select a certain proportion and quantity of normal repayment and overdue customers from the back end of the consumer finance platform according to the post-loan performance as modeling samples, collect the basic personal information of the sample customers when they apply for account registration, and obtain operational behavior from the monitoring software. point data;
[0104] S2: Data preprocessing, perform missing completion and outlier processing on the collected original data, and divide it into training set and test set according to the ratio of 7:3, and then normalize the training set and test set respectively;
[0105] S3: Determine the input and output data of neurons, and determine the optimal number of neurons in the hidden layer, and establish a...
Embodiment 2
[0198]The same parts of Embodiment 2 and Embodiment 1 will not be repeated. The difference is that in Step 1, a certain proportion and number of normal repayment and overdue customers are selected from the back end of the Internet financial platform according to the post-loan performance as modeling samples, and the samples are collected. The personal basic information when applying for customer account registration, and the embedded point data of operation behavior obtained in the monitoring software. The user's personal application information includes: mobile phone number, education background, marital status, work unit, address, contact information, basic personal information obtained from the credit report, credit transaction information, public information, and special record data; the buried point data Including the device behavior data and log data collected when the point is buried, the device behavior data includes: the number of logins to this platform, the number of...
PUM
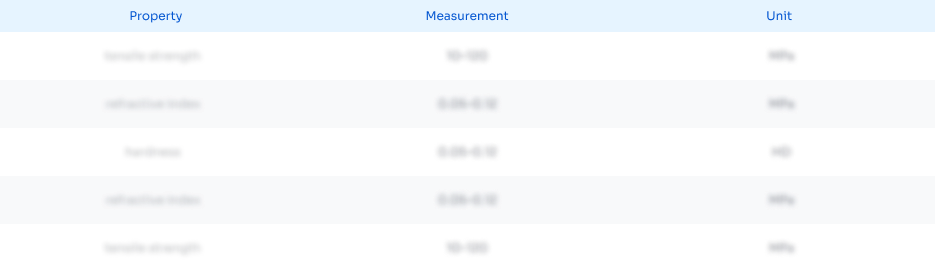
Abstract
Description
Claims
Application Information
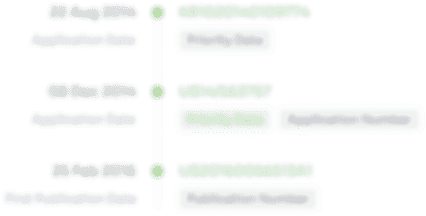
- R&D Engineer
- R&D Manager
- IP Professional
- Industry Leading Data Capabilities
- Powerful AI technology
- Patent DNA Extraction
Browse by: Latest US Patents, China's latest patents, Technical Efficacy Thesaurus, Application Domain, Technology Topic, Popular Technical Reports.
© 2024 PatSnap. All rights reserved.Legal|Privacy policy|Modern Slavery Act Transparency Statement|Sitemap|About US| Contact US: help@patsnap.com