Corn growth parameter active and passive remote sensing inversion method based on data augmentation and deep learning
A growth parameter and deep learning technology, applied in the field of remote sensing inversion, can solve the problem of low inversion accuracy
- Summary
- Abstract
- Description
- Claims
- Application Information
AI Technical Summary
Problems solved by technology
Method used
Image
Examples
Embodiment Construction
[0055] Reference will now be made in detail to the exemplary embodiments, examples of which are illustrated in the accompanying drawings. When the following description refers to the accompanying drawings, the same numerals in different drawings refer to the same or similar elements unless otherwise indicated. The implementations described in the following exemplary examples do not represent all implementations consistent with the present invention. Rather, they are merely examples of systems consistent with aspects of the invention as recited in the appended claims.
[0056] The active and passive remote sensing inversion method of corn LAI and biomass based on data augmentation and deep learning of the present invention is specifically a twin neural network (Gated Siamese Deep NeuralNetwork, GSDNN) based on data augmentation and gating mechanism. Active and Passive Remote Sensing Retrieval Method for Maize LAI and Biomass. This method mainly includes data preprocessing, da...
PUM
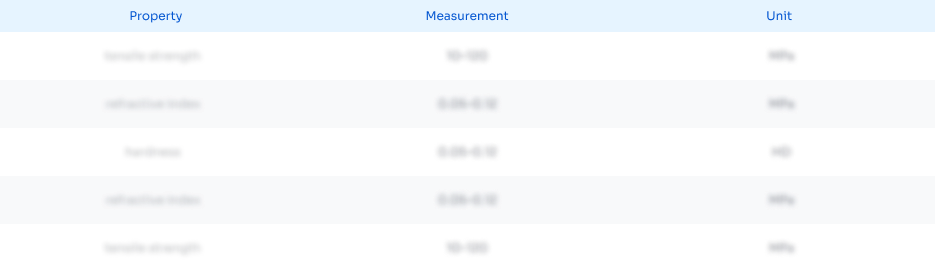
Abstract
Description
Claims
Application Information
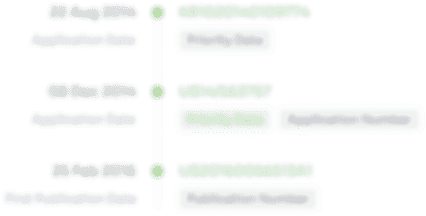
- R&D Engineer
- R&D Manager
- IP Professional
- Industry Leading Data Capabilities
- Powerful AI technology
- Patent DNA Extraction
Browse by: Latest US Patents, China's latest patents, Technical Efficacy Thesaurus, Application Domain, Technology Topic, Popular Technical Reports.
© 2024 PatSnap. All rights reserved.Legal|Privacy policy|Modern Slavery Act Transparency Statement|Sitemap|About US| Contact US: help@patsnap.com