Parallel cloud workflow scheduling method based on reinforcement learning strategy
A technology of reinforcement learning and workflow, applied in the field of cloud computing, can solve the problems that the neural network cannot handle the input information of dimension changes, cannot learn the knowledge of the task to be selected, and it is difficult to perceive dynamic changes, etc., so as to optimize the action selection strategy and increase Variety, the effect of improving optimization performance
- Summary
- Abstract
- Description
- Claims
- Application Information
AI Technical Summary
Problems solved by technology
Method used
Image
Examples
Embodiment Construction
[0048] The present invention will be described in detail below with reference to the accompanying drawings and examples.
[0049] In the prior art, the standard reinforcement learning algorithm DQN includes two parts, the Agent and the environment, and uses a neural network to approximate the action state value function. Among them, the interaction process between the Agent and the environment is as follows: In time step t, firstly, the Agent receives the state information of the environment (s t ), choose the action to be taken on the environment (a t ); Secondly, the action a t Act on the environment and get the environment's reward for the action (r t+1 ) and the next state after the environment update (s t+1 ); In the next time step t+1, first, judge whether the round is terminated. If the round is not over, the Agent obtains new environmental state information and performs new interactions with the environment. The specific framework is as follows figure 2 shown. D...
PUM
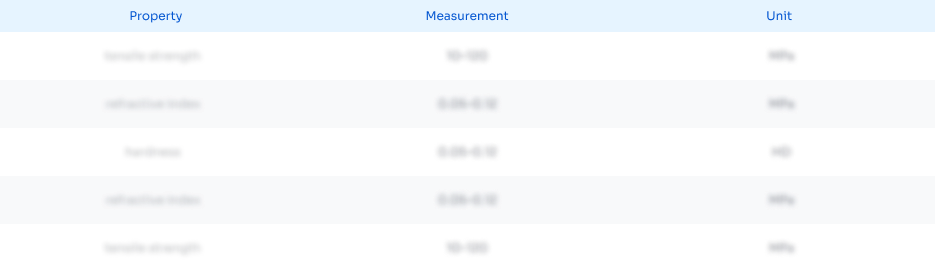
Abstract
Description
Claims
Application Information
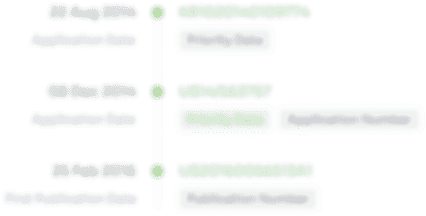
- R&D Engineer
- R&D Manager
- IP Professional
- Industry Leading Data Capabilities
- Powerful AI technology
- Patent DNA Extraction
Browse by: Latest US Patents, China's latest patents, Technical Efficacy Thesaurus, Application Domain, Technology Topic, Popular Technical Reports.
© 2024 PatSnap. All rights reserved.Legal|Privacy policy|Modern Slavery Act Transparency Statement|Sitemap|About US| Contact US: help@patsnap.com