Transformer fault diagnosis method based on deep learning
A transformer fault diagnosis method technology, applied in the direction of neural learning methods, transformer testing, instruments, etc., can solve the problems of limited diagnostic effect, cumbersome parameter setting, and slow convergence speed of neural network methods, so as to avoid the influence of subjective factors, The effect of high fault diagnosis accuracy and improvement of diagnosis accuracy
- Summary
- Abstract
- Description
- Claims
- Application Information
AI Technical Summary
Problems solved by technology
Method used
Image
Examples
Embodiment Construction
[0043] The present invention will be described in more detail below with reference to the accompanying drawings.
[0044] Flow chart of the present invention is attached figure 2 described, including the following steps:
[0045] Step 1, using the dissolved gas analysis method in oil to obtain the five gas H dissolved in the transformer oil 2 、CH 4 、C 2 h 2 、C 2 h 4 、C 2 h 6 concentration data;
[0046] Wherein, the transformer fault characteristic gas is H 2 、CH 4 、C 2 h 2 、C 2 h 4 、C 2 h 6 .
[0047]Step 2: In step 1, the original data is deduplicated, outlier detected, missing value filled and normalized. At the same time, the fault of the transformer is one_hot coded as a class label, and 80% of the various samples are taken. The training sample set, 20% of all kinds of samples constitute the test sample set;
[0048] In the fault data of transformers, due to factors such as human operation or sensor failure, repeated or abnormal data are collected, and...
PUM
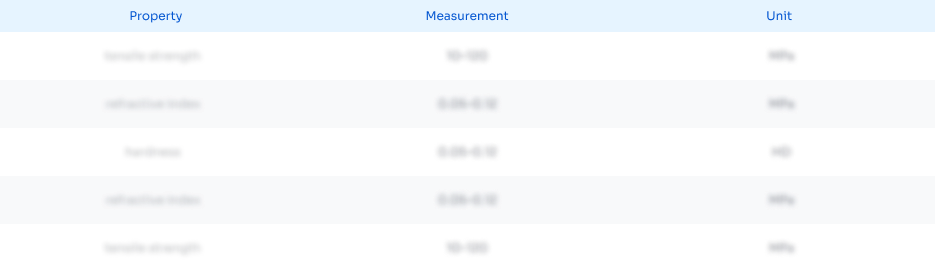
Abstract
Description
Claims
Application Information
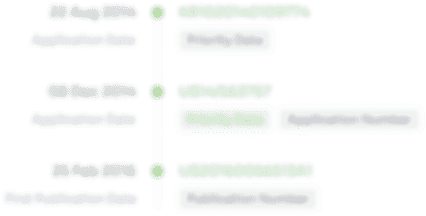
- R&D Engineer
- R&D Manager
- IP Professional
- Industry Leading Data Capabilities
- Powerful AI technology
- Patent DNA Extraction
Browse by: Latest US Patents, China's latest patents, Technical Efficacy Thesaurus, Application Domain, Technology Topic, Popular Technical Reports.
© 2024 PatSnap. All rights reserved.Legal|Privacy policy|Modern Slavery Act Transparency Statement|Sitemap|About US| Contact US: help@patsnap.com