Double-attention generative adversarial network for noise reduction and artifact removal of low-dose CT image
A CT image and image noise reduction technology, applied in the field of deep learning, can solve the problems of low density resolution of LDCT images, decreased X-ray penetration ability, incomplete projection data, etc., to solve the gradient disappearance or gradient explosion, and improve features Extraction ability and identification ability, low cost effect
- Summary
- Abstract
- Description
- Claims
- Application Information
AI Technical Summary
Problems solved by technology
Method used
Image
Examples
Embodiment Construction
[0061] In order to make the technical problems, technical solutions and beneficial effects to be solved by the present invention clearer, the present invention will be further described in detail below in conjunction with the accompanying drawings and embodiments. It should be understood that the specific embodiments described here are only used to explain the present invention, not to limit the present invention.
[0062] A dual-attention generative adversarial network for noise reduction and artifact removal in low-dose CT images. Using GAN network as the main framework, a dual-attention generative adversarial network is proposed to solve the artifact suppression technology in low-dose CT images. Program.
[0063] like Figure 1-2 As shown, the overall framework of the denoising network is divided into two subnetworks: a dual-attention generator subnetwork and a multi-scale discriminator subnetwork. First, input the LDCT image containing a lot of artifacts and noise into t...
PUM
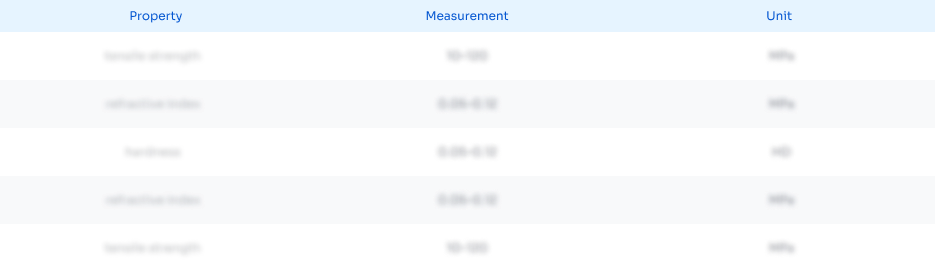
Abstract
Description
Claims
Application Information
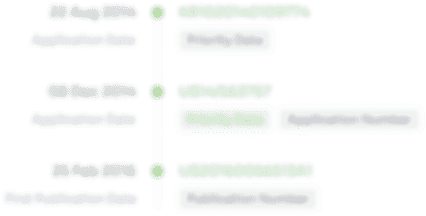
- R&D Engineer
- R&D Manager
- IP Professional
- Industry Leading Data Capabilities
- Powerful AI technology
- Patent DNA Extraction
Browse by: Latest US Patents, China's latest patents, Technical Efficacy Thesaurus, Application Domain, Technology Topic, Popular Technical Reports.
© 2024 PatSnap. All rights reserved.Legal|Privacy policy|Modern Slavery Act Transparency Statement|Sitemap|About US| Contact US: help@patsnap.com