Target detection model training method and device based on deep learning and storage medium
A technology for target detection and model training, applied in the field of deep learning target detection, can solve problems such as low confidence and wrong category labeling, achieve good performance, and improve accuracy and recall.
- Summary
- Abstract
- Description
- Claims
- Application Information
AI Technical Summary
Problems solved by technology
Method used
Image
Examples
Embodiment 1
[0028] First, refer to figure 1 Describe the deep learning-based target detection model training method of the present invention. Such as figure 1 As shown, the deep learning-based target detection model training method includes the following steps:
[0029] S101. Test the training images containing target labels, and obtain the targets contained in each training image. Specifically, when testing the training images containing target labels, the training images can be tested with the help of external public data sets (such as COCO data sets, etc.); it is also possible to directly input the training images into the trained target detection In the model, the training image is tested by the target detection model. At this time, the confidence threshold will be set lower to reduce the possibility of missing some targets. Specifically, the threshold can be set according to the recall rate of the algorithm, such as the confidence level corresponding to the recall rate of 99%.
...
Embodiment 2
[0037] When training an object detection model, it is necessary to calculate the ground truth (either the background or a certain category) for each location in the training image. In the anchor-based algorithm, the intersection over union ratio (Intersection over Union, iou) between the bounding box (anchor) of the target label and the prediction box is usually calculated. If the iou is greater than the preset threshold, it is set as a positive sample, otherwise it is Negative samples. Due to the influence of factors such as the translation and size of the target, the iou of some targets and anchors may be smaller than the preset threshold. If the positive and negative samples are divided directly based on the iou, it will lead to missed detection of positive samples. In the anchor-free algorithm, when it is judged that the size of the target is within the scale range of the layer network, and there is a feature point in the bounding box marked by the target, the feature poin...
Embodiment 3
[0043] In this embodiment, a further design is made on the basis of the second embodiment, which takes the similarity of categories into consideration when calculating the classification loss of positive samples. For example, when the target is a pedestrian, the probability of the cyclist being judged as the background will be lower.
[0044] Specifically, first, set the similarity matrix of each category, the value of each element of the main diagonal in the similarity matrix is 1, and the other elements take values in the interval [0,1] according to the similarity of the category, and the categories are similar The larger the degree, the smaller the value of the element to reduce the interaction between elements. Then, the classification loss of each category is calculated for each feature point, and finally the product is multiplied by the similarity matrix and summed to obtain the classification loss of the positive sample. For example, the similarity matrix looks l...
PUM
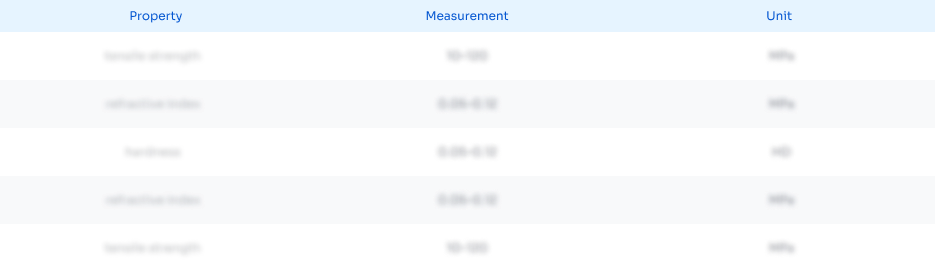
Abstract
Description
Claims
Application Information
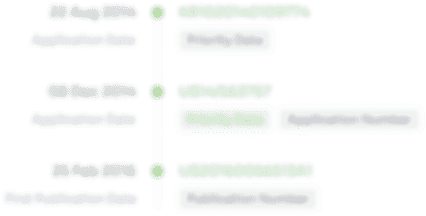
- R&D Engineer
- R&D Manager
- IP Professional
- Industry Leading Data Capabilities
- Powerful AI technology
- Patent DNA Extraction
Browse by: Latest US Patents, China's latest patents, Technical Efficacy Thesaurus, Application Domain, Technology Topic, Popular Technical Reports.
© 2024 PatSnap. All rights reserved.Legal|Privacy policy|Modern Slavery Act Transparency Statement|Sitemap|About US| Contact US: help@patsnap.com