Low-overhead household garbage classification method based on dense convolutional network
A convolutional network and domestic waste technology, applied in neural learning methods, biological neural network models, image data processing, etc., can solve the problems of limited model resources, low recognition accuracy, difficult promotion, etc., to slow down the problem of gradient disappearance, Enhance the effect of feature information and low precision
- Summary
- Abstract
- Description
- Claims
- Application Information
AI Technical Summary
Problems solved by technology
Method used
Image
Examples
Embodiment Construction
[0029] If no specific experimental steps or conditions are indicated in the examples, it can be carried out according to the operation or conditions of the conventional experimental steps described in the literature in this field. The reagents or instruments used, whose manufacturers are not indicated, are all commercially available conventional reagent products.
[0030] Such as Figure 1-6 as shown, figure 1 It is a model frame diagram, which is divided into three parts: data preprocessing module, dense convolutional network module, output classification and model evaluation module. The specific process is as follows:
[0031] (1) Data preprocessing module: first download the Trashnet open source data set, which consists of 2527 pictures of six categories of garbage, that is, N is 2527, namely: cardboard, glass bottles, metal cans, paper, plastic, other garbage . All scaled to 224×224 size using PIL’s Image module bilinear interpolation method. Use the rotate method of...
PUM
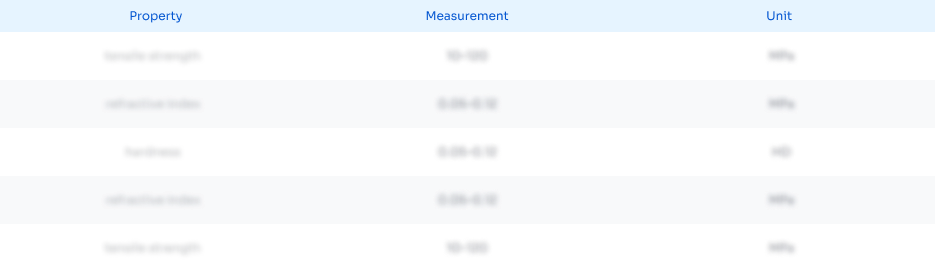
Abstract
Description
Claims
Application Information
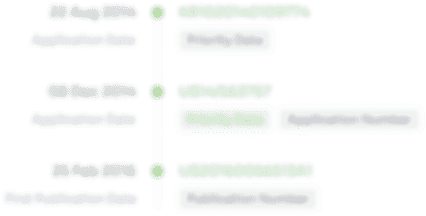
- R&D Engineer
- R&D Manager
- IP Professional
- Industry Leading Data Capabilities
- Powerful AI technology
- Patent DNA Extraction
Browse by: Latest US Patents, China's latest patents, Technical Efficacy Thesaurus, Application Domain, Technology Topic, Popular Technical Reports.
© 2024 PatSnap. All rights reserved.Legal|Privacy policy|Modern Slavery Act Transparency Statement|Sitemap|About US| Contact US: help@patsnap.com