Medium and long term runoff ensemble forecasting method based on multi-model combination
An ensemble forecast, medium and long-term technology, applied in computational models, biological models, neural learning methods, etc. The effect of improving accuracy and reference value
- Summary
- Abstract
- Description
- Claims
- Application Information
AI Technical Summary
Problems solved by technology
Method used
Image
Examples
Embodiment
[0047] This embodiment provides a method for ensemble forecasting of medium and long-term runoff based on multi-model combination, including the following steps:
[0048] S1, climate system index-runoff correlation analysis: Select multiple climate system index historical data and historical runoff data of the watershed to be forecasted, and use the correlation analysis method to calculate the correlation coefficient between runoff and climate system index; select the correlation coefficient The top 20 items with the largest absolute value are used as primary selection factors to form a primary selection factor matrix;
[0049] S2, extract key influencing factors: further perform dimension reduction processing on the primary factor matrix obtained in step S1, extract key influencing factors affecting the runoff process, and generate a key influencing factor matrix;
[0050] S3. Construction of impact factor-runoff data set: normalize the key impact factor matrix obtained in st...
specific Embodiment
[0081] This example proposes a medium- and long-term runoff ensemble forecasting method based on multi-model combination, such as figure 1 As shown, firstly, the correlation relationship between 130 climate system indices and historical runoff is analyzed, and the primary factor matrix is extracted from it, and then the dimensionality of the primary factor matrix is reduced by the principal component analysis method to obtain the key influencing factor matrix. Then it is zero-mean normalized, and then combined with historical runoff data to construct a sample model data set. Divide the sample model data set into two categories, one is the training set and the other is the test set, and use the training set to train the sample models based on SVR, KNN and BP neural network respectively. The training process of the three sample models is as follows:
[0082] The process of training the SVR model is as follows figure 2 As shown, the particle swarm optimization algorithm is ...
PUM
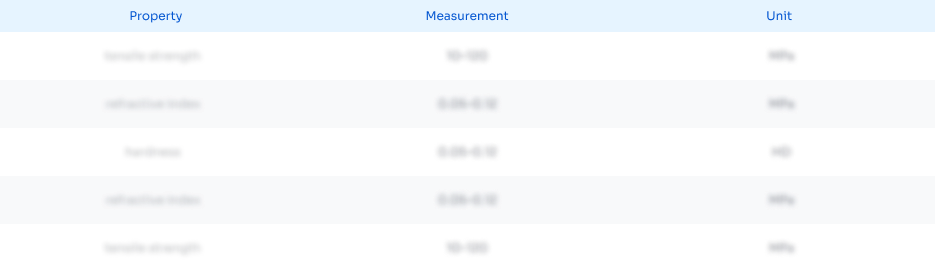
Abstract
Description
Claims
Application Information
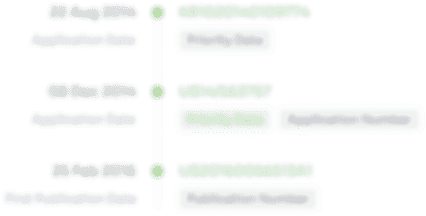
- R&D Engineer
- R&D Manager
- IP Professional
- Industry Leading Data Capabilities
- Powerful AI technology
- Patent DNA Extraction
Browse by: Latest US Patents, China's latest patents, Technical Efficacy Thesaurus, Application Domain, Technology Topic.
© 2024 PatSnap. All rights reserved.Legal|Privacy policy|Modern Slavery Act Transparency Statement|Sitemap