Multi-branch target detection method based on traffic scene
A traffic scene, target detection technology, applied in the direction of instruments, biological neural network models, character and pattern recognition, etc., can solve the problems of accumulating errors, difficult to accurately detect small targets, destroying the original structure of small targets, etc., and achieve high operating efficiency. , The effect of meeting real-time detection requirements
- Summary
- Abstract
- Description
- Claims
- Application Information
AI Technical Summary
Problems solved by technology
Method used
Image
Examples
Embodiment Construction
[0030] The technical solutions in the embodiments of the present invention will be described clearly and in detail below with reference to the drawings in the embodiments of the present invention. The described embodiments are only some of the embodiments of the invention.
[0031] The technical scheme that the present invention solves the problems of the technologies described above is:
[0032] The network structure of the present invention is attached as figure 1 shown, with figure 2 The specific parameters of each layer of the network are given.
[0033] Specific steps:
[0034] Step S1: Obtain high-definition photos taken at traffic intersections to construct relevant data sets, classify and label traffic scene images, generate corresponding category labels, and divide training sets and test sets for subsequent network training and testing;
[0035] Step S2: The bounding box regression process is calculated by the following formula:
[0036] first pass
[0037] t ...
PUM
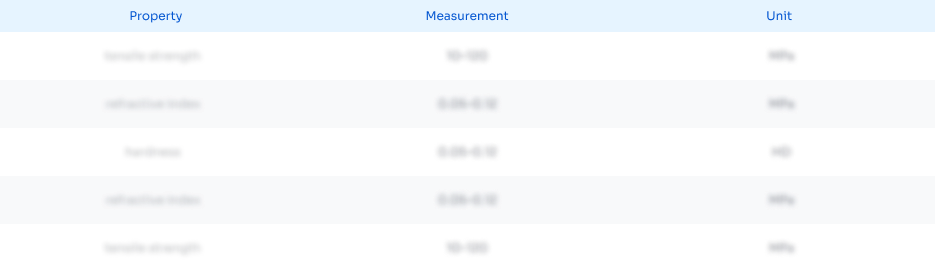
Abstract
Description
Claims
Application Information
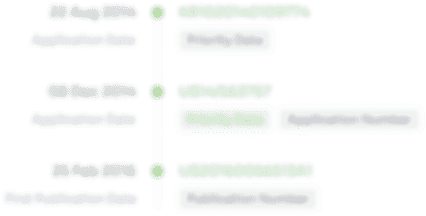
- R&D Engineer
- R&D Manager
- IP Professional
- Industry Leading Data Capabilities
- Powerful AI technology
- Patent DNA Extraction
Browse by: Latest US Patents, China's latest patents, Technical Efficacy Thesaurus, Application Domain, Technology Topic, Popular Technical Reports.
© 2024 PatSnap. All rights reserved.Legal|Privacy policy|Modern Slavery Act Transparency Statement|Sitemap|About US| Contact US: help@patsnap.com