Welding spot quality detection method based on a deep learning target detection algorithm and an implementation system thereof
A target detection algorithm and solder joint quality technology, applied in the field of image processing, can solve problems such as increased calculation amount and time consumption, complex system model, influence, etc., to achieve better expression of image information, increase positioning accuracy, and increase robustness. Effect
- Summary
- Abstract
- Description
- Claims
- Application Information
AI Technical Summary
Problems solved by technology
Method used
Image
Examples
Embodiment 1
[0078] A solder joint quality detection method based on deep learning target detection algorithm, such as figure 1 shown, including the following steps:
[0079] (1) From the Hisense optical module production line, collect images of the solder joints of the device ROSA or TOSA welded to the circuit board. The data set has a total of 4598 pictures, and all the pictures are marked with data, and each solder joint is framed with a rectangular frame, and the judgment is made manually Finally, record the quality of solder joints and the position of the rectangular frame. Normalize the size of all pictures so that the size of all pictures becomes (600×800), and divide the marked data set into 4098 training sets and 500 test sets. The 4098 marked pictures in the training set are subjected to data enhancement, that is, the pictures are rotated at random angles, scaled, flipped and other operations to achieve the purpose of enhancing the data type.
[0080] (2) feature extraction to ...
Embodiment 2
[0085] According to a kind of solder joint quality detection method based on deep learning target detection algorithm described in embodiment 1, its difference is:
[0086] In the step (1), the data is collected and pre-processed, including steps as follows:
[0087] A. Use a microscope with a light source to take pictures of devices with a resolution of 600-1200 including solder joints;
[0088] B. For each solder joint in each picture, mark its position with a box of appropriate size that completely covers the solder joint. The position of each solder joint is represented by four parameters, namely (x, y, w, h) ;Take the lower left corner of the picture as the origin, the left border of the picture is the x-axis, and the lower border of the picture is the y-axis to establish a coordinate system, (x, y) indicates the coordinate position of the upper left corner of the box in the coordinate system, and w indicates the box The width of h represents the length of the box;
[0...
Embodiment 3
[0092] According to a kind of solder joint quality detection method based on deep learning target detection algorithm described in embodiment 1, its difference is:
[0093] In step (2), extracting features from the preprocessed picture in step (1), including:
[0094] Image features are extracted through the deep neural network model ResNet50. The deep neural network model ResNet50 has a total of 50 layers, including 49 convolutional layers and 1 fully connected layer. The 49-layer convolutional layer includes 5 convolutional parts, and the fourth convolutional part The size of the output feature map is 38×50; the deep neural network model ResNet50 is not only easy to optimize the network, but also can avoid the problem of performance degradation caused by too deep network layers. The definition of the feature map output by the convolutional layer is shown in formula (I):
[0095]
[0096] In formula (Ⅰ), Indicates the j-th feature map of the l-th layer, Represents the...
PUM
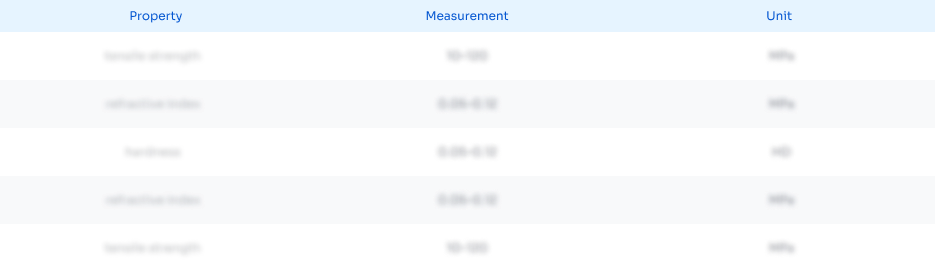
Abstract
Description
Claims
Application Information
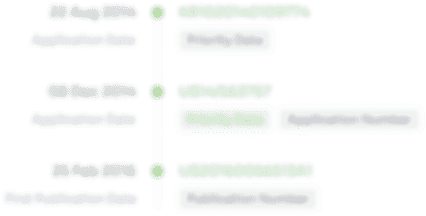
- R&D Engineer
- R&D Manager
- IP Professional
- Industry Leading Data Capabilities
- Powerful AI technology
- Patent DNA Extraction
Browse by: Latest US Patents, China's latest patents, Technical Efficacy Thesaurus, Application Domain, Technology Topic, Popular Technical Reports.
© 2024 PatSnap. All rights reserved.Legal|Privacy policy|Modern Slavery Act Transparency Statement|Sitemap|About US| Contact US: help@patsnap.com