Tranform-based quotation network classification model establishment and classification of graph convolution network
A convolutional network and classification model technology, applied in the field of citation network classification model establishment and classification, can solve the problem that convolutional network cannot perform deep learning, achieve the advantages of time complexity and space complexity, increase time complexity and space complexity, the effect of avoiding over-smoothing of features
- Summary
- Abstract
- Description
- Claims
- Application Information
AI Technical Summary
Problems solved by technology
Method used
Image
Examples
Embodiment Construction
[0057] First, the technical terms that appear in the present invention are explained:
[0058] Citation Network: A dataset consisting of papers, authors and their citation relationships. These papers / authors (nodes) are connected to each other through citation relationships (edges), and these papers / authors have a corresponding category label, which is a graph-structured dataset, that is, the nodes are organized in a many-to-many manner . A general citation network is organized in two parts: features and graphs, that is, their connection relationships are organized into a graph, usually using an adjacency matrix or a dictionary for storage. In actual use, if it is stored in a dictionary, it is generally necessary to It is further processed into the form of adjacency matrix, and the other part is the feature of the node, which is generally stored as a one-dimensional vector. Each dimension of the vector corresponds to a word in the dictionary, that is, the node itself is descr...
PUM
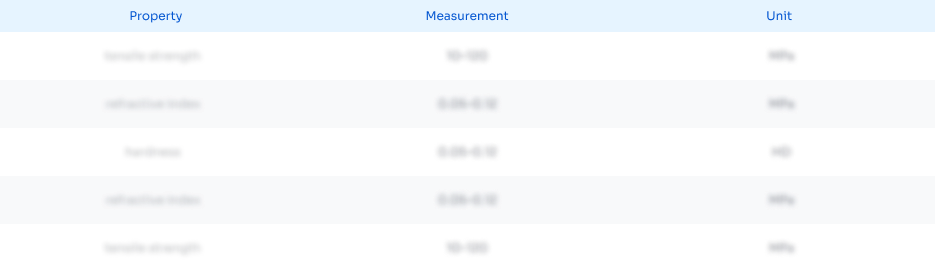
Abstract
Description
Claims
Application Information
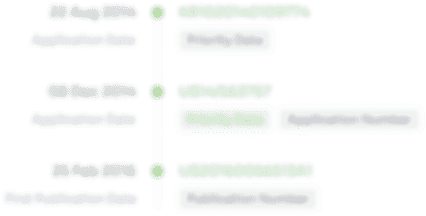
- R&D Engineer
- R&D Manager
- IP Professional
- Industry Leading Data Capabilities
- Powerful AI technology
- Patent DNA Extraction
Browse by: Latest US Patents, China's latest patents, Technical Efficacy Thesaurus, Application Domain, Technology Topic, Popular Technical Reports.
© 2024 PatSnap. All rights reserved.Legal|Privacy policy|Modern Slavery Act Transparency Statement|Sitemap|About US| Contact US: help@patsnap.com