Rolling bearing fault detection method based on multilayer residual network model
A technology of network model and rolling bearing, which is applied in biological neural network model, testing of mechanical components, testing of machine/structural components, etc., can solve problems such as economic loss, high accuracy, and inconsistency, and achieve good robustness, The effect of high classification accuracy and fast feature extraction speed
- Summary
- Abstract
- Description
- Claims
- Application Information
AI Technical Summary
Problems solved by technology
Method used
Image
Examples
Embodiment 1
[0062] Embodiment 1: with reference to attached figure 1 A rolling bearing fault detection method based on a multi-layer residual network model is shown, including the steps
[0063]Step1. Use the sensor to collect and extract the vibration signal of the bearing
[0064] Use an acceleration sensor to measure the vibration signals of the experimental bearing under normal conditions and under four conditions: inner ring fault, outer ring fault, and rolling element fault, and obtain sample point data including vibration signals in normal state and fault state;
[0065] Step2. Input the vibration signal collected by the sensor into the residual neural network, and repeatedly use the residual network to iterate the vibration signal between different convolutional layers
[0066] Step201. Input the vibration signal extracted in Step 1 into the convolution layer 1 for feature extraction;
[0067] Wherein: 1 input channel is arranged in the described convolution layer 1, 4 output ch...
Embodiment 2
[0100] Embodiment 2: as Figure 2-3 As shown, in order to prove the effectiveness of this method, the vibration data in the United States is used from the open data set of Case Western Reserve University in the United States:
[0101] (1) In this experiment, the bearings at the drive end of the motor and the fan end are used as the diagnostic objects, and single-point damage is introduced on the inner ring, rolling element, and outer ring of the test bearings by EDM to simulate three types of bearing failures. The fault damage scales are 0.007inch, 0.014inch and 0.021inch respectively, and then under similar working conditions (equal load, close speed), the fault signal is collected by the acceleration sensor on the upper side of the motor drive end, and the sampling frequency is 12kHz. According to the location and size of the bearing fault, the bearing categories are divided into ten categories, with 1024 data points as a sample, and 1600 samples are selected for each catego...
Embodiment 3
[0107] Embodiment 3: use faulty bearing to carry out comparative test to the fault detection method of different rolling bearings below, further the detection method of the present invention is verified:
[0108] Step 1: Select a set of rolling bearing data, and the failure conditions of the bearings are shown in Table 1:
[0109] Table 1: Fault information of rolling bearings
[0110]
[0111] Step 2: Use time-frequency feature+SV, ED+SV, wavelet+SV, CNN-SV and the multi-layer residual network model of the present invention to detect the faulty bearing described in step 1 respectively, and detect The obtained results are compared with the data in Table 1 above, and the comparison results of the time-consuming and accuracy of each detection method are shown in Table 2:
[0112] Table 2: Comparison of accuracy rates of different methods
[0113]
[0114] As can be seen from the results in Table 2, under the same conditions, the diagnostic method based on the multi-layer...
PUM
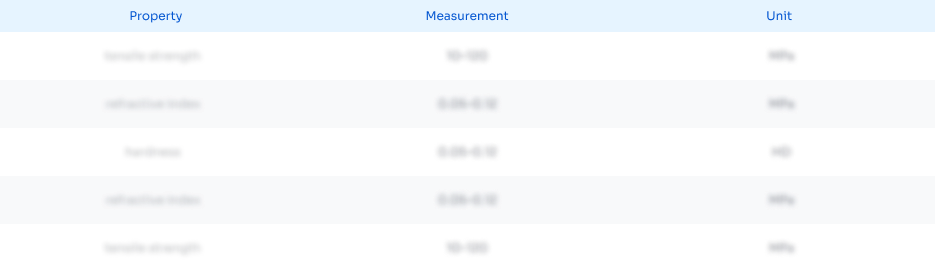
Abstract
Description
Claims
Application Information
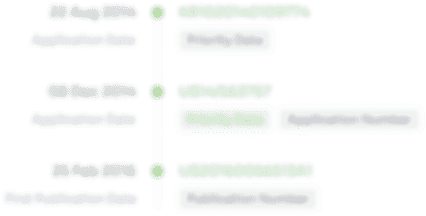
- R&D Engineer
- R&D Manager
- IP Professional
- Industry Leading Data Capabilities
- Powerful AI technology
- Patent DNA Extraction
Browse by: Latest US Patents, China's latest patents, Technical Efficacy Thesaurus, Application Domain, Technology Topic, Popular Technical Reports.
© 2024 PatSnap. All rights reserved.Legal|Privacy policy|Modern Slavery Act Transparency Statement|Sitemap|About US| Contact US: help@patsnap.com