Bioactive peptide prediction method based on deep convolutional neural network
A bioactive peptide, neural network technology, applied in neural learning methods, biological neural network models, neural architectures, etc.
- Summary
- Abstract
- Description
- Claims
- Application Information
AI Technical Summary
Problems solved by technology
Method used
Image
Examples
Embodiment 1
[0047] 1. Benchmark comparison between AAPred-CNN and existing prediction methods
[0048] Experimental setup. To evaluate the effectiveness of AAPred-CNN, benchmark datasets (including S main and S NT15 ) for independent testing. Note that independent testing can assess model generalization when given unseen (new) samples, which is sufficient for model comparison. We report the performance comparison and analysis of AAPred-CNN with the state-of-the-art models. image 3 The experimental results are summarized.
[0049] AAPred-CNN outperforms the current state-of-the-art models. like image 3 As shown, in the main dataset, AAPred-CNN performs 2.58%, 2.75%, 9.06%, 5.77% higher than TargetAntiAngio in terms of ACC, BACC, SP, and MCC, respectively. Similarly, in the NT15 dataset, AAPred-CNN outperforms the AntiAngioPred method by 5.00%, 5.68%, 36.84% and 13.69% on ACC, BACC, SP and MCC, respectively. Both comparative results show the comprehensive advantages of the propose...
Embodiment 2
[0051] 2. Research on the influence of AAPred-CNN hyperparameters on performance
[0052] Experimental setup. To investigate which factors play an important role in AAPred-CNN, we selected hyperparameters such as learning rate, batch size, and embedding dimension to study the impact on the performance of AAPred-CNN. For convenience, we only select commonly used candidate hyperparameters for study. Specifically, the learning rate is chosen from 0.0005 to 0.0030, and the batch size is chosen from 16 to 128. Both the number of filters and the embedding dimension range from 16 to 512. Compare the result as Figure 4-5 shown.
[0053] For the two evaluation datasets, the performance of AAPred-CNN is relatively stable when the hyperparameters are within a certain range. from Figure 4-5 It can be seen that when the learning rate is about 0.0001, the batch size is about 32, the number of filters is about 128, and the embedding dimension is about 128, the model can achieve the b...
Embodiment 3
[0055] 3. Research on the influence of training data volume on model performance
[0056] Experimental setup. As mentioned above, it is counterintuitive that deep learning models achieve superior performance despite only being given a few training samples. Therefore, it is necessary to investigate to what extent the performance of deep learning models depends on the amount of data in order to further discuss why deep learning models can work well in such few example scenarios. for S main and S NT15 . We keep the test set unchanged and use different proportions of the training set to build the evaluation model. Specifically, from 0 to 100%, every 10% is selected as an interval to randomly select the corresponding proportion of the training set. The results of the comparative experiments are as Figure 6-7 shown.
[0057] The larger the proportion of training samples, the better the model performance. Figure 6-7 The comparison results in [20] show that the overall perfo...
PUM
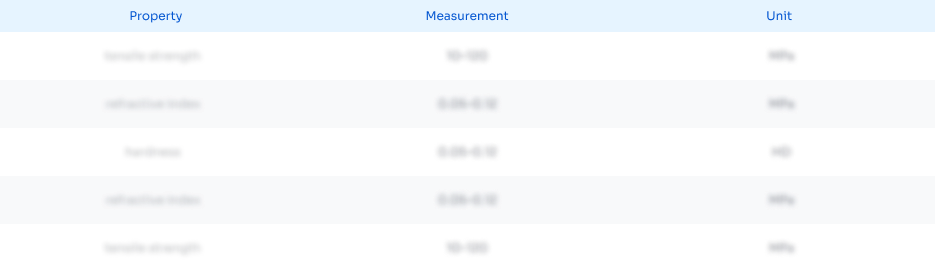
Abstract
Description
Claims
Application Information
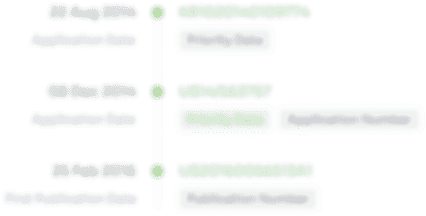
- R&D Engineer
- R&D Manager
- IP Professional
- Industry Leading Data Capabilities
- Powerful AI technology
- Patent DNA Extraction
Browse by: Latest US Patents, China's latest patents, Technical Efficacy Thesaurus, Application Domain, Technology Topic, Popular Technical Reports.
© 2024 PatSnap. All rights reserved.Legal|Privacy policy|Modern Slavery Act Transparency Statement|Sitemap|About US| Contact US: help@patsnap.com