Medium-voltage circuit breaker fault diagnosis method based on deep learning and intelligent optimization
A circuit breaker failure and intelligent optimization technology, applied in the direction of neural learning methods, kernel methods, instruments, etc., can solve the problems of increasing circuit breaker downtime and maintenance and overhaul time, poor generalization ability, slow diagnosis speed, etc., and achieve the elimination of local maximum Excellent phenomenon, excellent representation ability, and the effect of improving accuracy
- Summary
- Abstract
- Description
- Claims
- Application Information
AI Technical Summary
Problems solved by technology
Method used
Image
Examples
Embodiment
[0039] Example: for a medium voltage circuit breaker in the common type of fault categories, with reference to Figure 3-6 As shown in a medium voltage circuit breaker intelligent diagnostic method based on CNN-QPSO-SVM, comprising the steps of:
[0040] S1: an acceleration sensor vibration acceleration parameter recording circuit breaker / off process, the sampling frequency is 10kHz. An acceleration sensor mounted on the circuit breaker housing, for collecting the vertical vibration signals, a total of 7 sets of vibration signals collected data, comprising: a normal state; tripping the closing electromagnet blocked; spindle plug; 4 principal axle blockage fault types . Fault Type and number as shown in Table 1.
[0041] Table 1 Class 4 breaker common mechanical faults ID
[0042]
[0043] S2: The training data and test data is normalized, the normalized using the following formula:
[0044]
[0045] xi Original data sample, x max X i The maximum value, x min X i Minimum. In t...
PUM
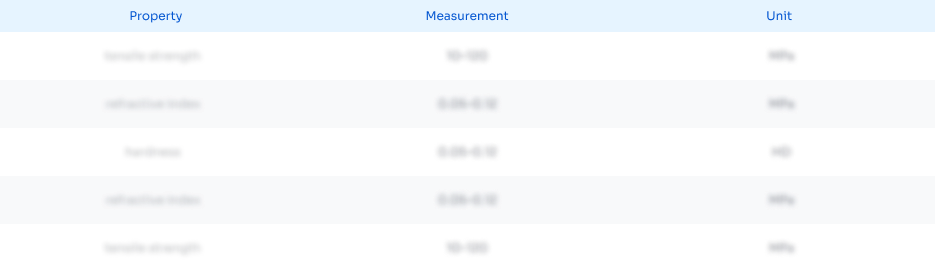
Abstract
Description
Claims
Application Information
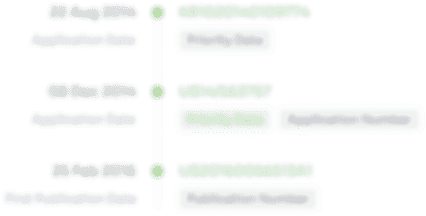
- R&D Engineer
- R&D Manager
- IP Professional
- Industry Leading Data Capabilities
- Powerful AI technology
- Patent DNA Extraction
Browse by: Latest US Patents, China's latest patents, Technical Efficacy Thesaurus, Application Domain, Technology Topic, Popular Technical Reports.
© 2024 PatSnap. All rights reserved.Legal|Privacy policy|Modern Slavery Act Transparency Statement|Sitemap|About US| Contact US: help@patsnap.com