Non-small cell lung cancer subtype classification system based on multi-view deep learning
A non-small cell lung cancer and deep learning technology, applied in the field of non-small cell lung cancer subtype classification system, can solve problems such as difficulty in describing the whole picture of tumors, patient treatment delays, accuracy doubts, etc., to improve model learning efficiency and classification accuracy The effect of improving the rate, improving generalization ability, and high model classification accuracy
- Summary
- Abstract
- Description
- Claims
- Application Information
AI Technical Summary
Problems solved by technology
Method used
Image
Examples
Embodiment 1
[0061] First, for each CT image, the data containing only the lung field is obtained through preprocessing methods such as rough segmentation and edge cleaning, in preparation for saving multi-view data later.
[0062] The data used comes from the Cancer Imaging Archives (NCI-TCIA) under the National Cancer Institute of the United States. For each lung CT image, the data containing only the lung field is obtained. The specific method is: based on the scanning slice thickness of different CT machines, approximate Interpolation is performed using a point sampling method, which interpolates a matrix by copying adjacent pixels. Through this method, each voxel is normalized to 1mm×1mm×1mm, which ensures that the original size of the lesion is restored and facilitates the training of the multi-view model.
[0063] Based on the pixel value of CT data, normalize each piece of data with a window width of 2000 and a window level of 0 (unit: Hu), and use -400 as the threshold to perform ...
PUM
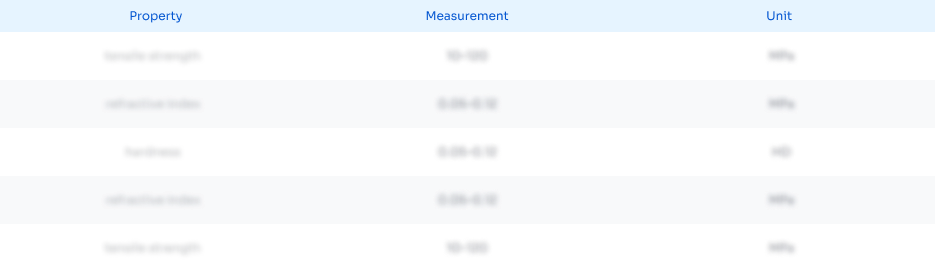
Abstract
Description
Claims
Application Information
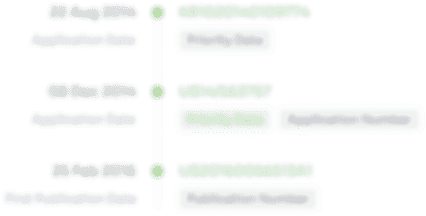
- R&D Engineer
- R&D Manager
- IP Professional
- Industry Leading Data Capabilities
- Powerful AI technology
- Patent DNA Extraction
Browse by: Latest US Patents, China's latest patents, Technical Efficacy Thesaurus, Application Domain, Technology Topic, Popular Technical Reports.
© 2024 PatSnap. All rights reserved.Legal|Privacy policy|Modern Slavery Act Transparency Statement|Sitemap|About US| Contact US: help@patsnap.com