Target detection method based on self-supervised generative adversarial learning background modeling
A technology of target detection and background modeling, applied in neural learning methods, inference methods, biological neural network models, etc., can solve the problems of brightness camera moving target detection algorithm difficulty, brightness changing background dynamic camera movement, difficulty, etc. Reduce dependence, widely use scenarios and practical application value, improve the effect of the effect
- Summary
- Abstract
- Description
- Claims
- Application Information
AI Technical Summary
Problems solved by technology
Method used
Image
Examples
Embodiment Construction
[0022] The embodiments of the present invention will be described in further detail below with reference to the accompanying drawings.
[0023] A target detection method based on self-supervised generative adversarial learning background modeling, including the following steps:
[0024] Step 1. Use the camera in the environment to collect images, and label the foreground targets to obtain corresponding labels, and construct a dataset S.
[0025] Each sample consists of an image and a corresponding foreground target mask label. The foreground target mask label is a binary image of the same size as the original image. The pixels of the foreground target are marked as 1 and the background pixels are marked as 0.
[0026] Step 2. Select a data subset S that only contains background information images from the data set S b .
[0027] Step 3. Construct a generative adversarial network consisting of a generative network G and a discriminant network D, and use the data set S using s...
PUM
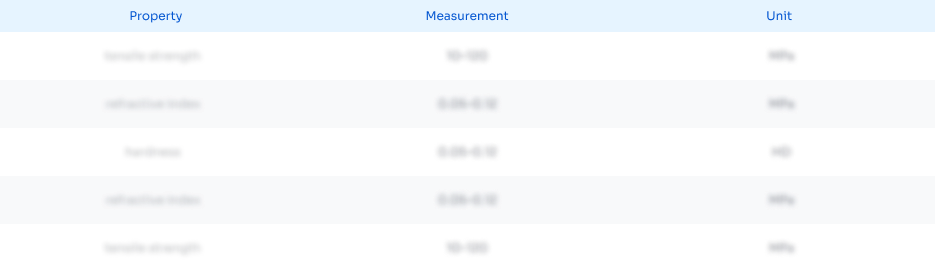
Abstract
Description
Claims
Application Information
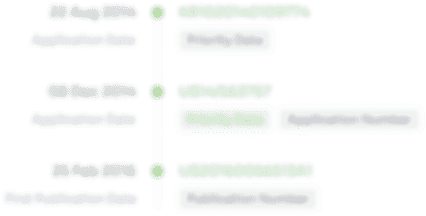
- R&D Engineer
- R&D Manager
- IP Professional
- Industry Leading Data Capabilities
- Powerful AI technology
- Patent DNA Extraction
Browse by: Latest US Patents, China's latest patents, Technical Efficacy Thesaurus, Application Domain, Technology Topic, Popular Technical Reports.
© 2024 PatSnap. All rights reserved.Legal|Privacy policy|Modern Slavery Act Transparency Statement|Sitemap|About US| Contact US: help@patsnap.com