Milling cutter wear state real-time monitoring method based on deep convolutional neural network
A technology of tool wear and CNC machine tools, which is applied in the direction of manufacturing tools, measuring/indicating equipment, metal processing machinery parts, etc., can solve the problems of large deviation in predicting critical states and low prediction accuracy of tool wear values, and achieve improved prediction and The effect of recognition accuracy, improvement of monitoring efficiency, and reduction of subjectivity
- Summary
- Abstract
- Description
- Claims
- Application Information
AI Technical Summary
Problems solved by technology
Method used
Image
Examples
Embodiment Construction
[0041] The present invention will be described in further detail below in conjunction with the accompanying drawings and examples of implementation, but it should be understood that the examples are used to explain the present invention, not to limit the present invention.
[0042] The invention provides a real-time monitoring method for milling tool wear of CNC machine tools based on a deep convolutional neural network. The provided monitoring method is completed in the following steps: First, obtain multi-source heterogeneous state data signals (force signal, vibration, etc.) from sensors. signal, power signal, acoustic emission signal) and related information of the tool wear life cycle, data cleaning, compression sensing, noise processing, and normalization processing are performed on the data; secondly, the labeled data set is used to train the tool wear value Regression model, constantly correcting network model parameters, and visualizing the influence of parameter chang...
PUM
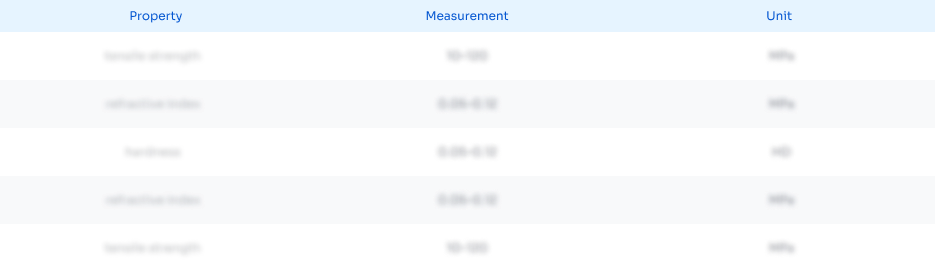
Abstract
Description
Claims
Application Information
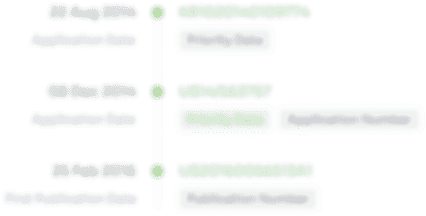
- R&D
- Intellectual Property
- Life Sciences
- Materials
- Tech Scout
- Unparalleled Data Quality
- Higher Quality Content
- 60% Fewer Hallucinations
Browse by: Latest US Patents, China's latest patents, Technical Efficacy Thesaurus, Application Domain, Technology Topic, Popular Technical Reports.
© 2025 PatSnap. All rights reserved.Legal|Privacy policy|Modern Slavery Act Transparency Statement|Sitemap|About US| Contact US: help@patsnap.com