Large-scale point cloud semantic segmentation method based on lightweight neural network
A semantic segmentation and neural network technology, applied in biological neural network models, neural learning methods, neural architectures, etc., can solve the problem of high computational complexity of semantic segmentation methods, achieve simplified point cloud semantic segmentation networks, reduce time complexity, The effect of reducing training time
- Summary
- Abstract
- Description
- Claims
- Application Information
AI Technical Summary
Problems solved by technology
Method used
Image
Examples
Embodiment Construction
[0027] The specific implementation manners of the present invention are described below, so that those skilled in the art can implement with reference to the description.
[0028] Such as figure 1 , 2 , the present invention provides a 3D point cloud semantic segmentation method based on a lightweight neural network, comprising:
[0029] S1. Use the 3D point cloud training set to train the semantic segmentation neural network model, and the training samples are 3D lidar point clouds {P i |i=1,2,...,n}, where each point P i is a vector (x, y, z, int, ret, num) representing the original (x, y, z) coordinates, intensity, number of returns, and number of returns of the lidar data, respectively, and corresponds to a label y i , representing the real semantic category.
[0030] Perform rasterization, sampling, and normalization preprocessing on the point cloud dataset to establish a training set.
[0031] The data set used in the present invention is a remote sensing three-dime...
PUM
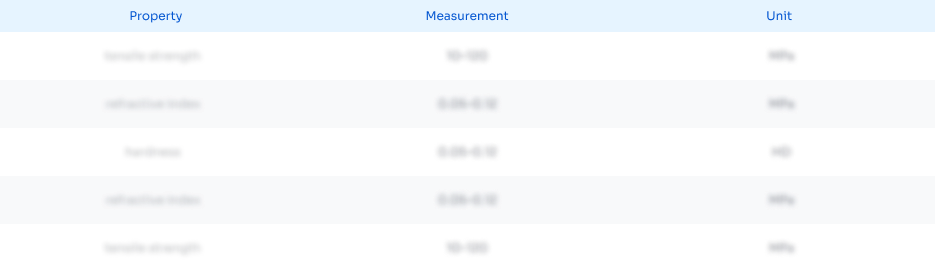
Abstract
Description
Claims
Application Information
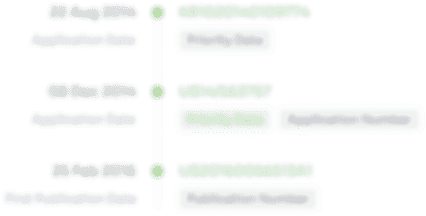
- R&D Engineer
- R&D Manager
- IP Professional
- Industry Leading Data Capabilities
- Powerful AI technology
- Patent DNA Extraction
Browse by: Latest US Patents, China's latest patents, Technical Efficacy Thesaurus, Application Domain, Technology Topic, Popular Technical Reports.
© 2024 PatSnap. All rights reserved.Legal|Privacy policy|Modern Slavery Act Transparency Statement|Sitemap|About US| Contact US: help@patsnap.com