Pipeline corrosion defect prediction method based on optimized neural network
A neural network and prediction method technology, applied in the field of oil and gas pipelines, can solve the problems of neural network model instability, reduce algorithm convergence speed, reduce training efficiency, etc., achieve the goals of increasing learning rate and accuracy, improving stability, and reducing detection frequency Effect
- Summary
- Abstract
- Description
- Claims
- Application Information
AI Technical Summary
Problems solved by technology
Method used
Image
Examples
Embodiment Construction
[0041] In order to enable those skilled in the art to better understand the technical solutions in the present application, the technical solutions in the embodiments of the present application will be clearly and completely described below in conjunction with the drawings in the embodiments of the present application. Obviously, the described The embodiments are only some of the embodiments of the present application, but not all of them. Based on the embodiments in this application, all other embodiments obtained by persons of ordinary skill in the art without creative efforts shall fall within the scope of protection of this application.
[0042] refer to figure 1 , a method for predicting pipeline corrosion defects based on an optimized neural network provided by an embodiment of the present invention includes the following steps:
[0043] Step 1. Collect the corrosion defect information in the pipeline and the transport medium conditions of the pipeline to which it belo...
PUM
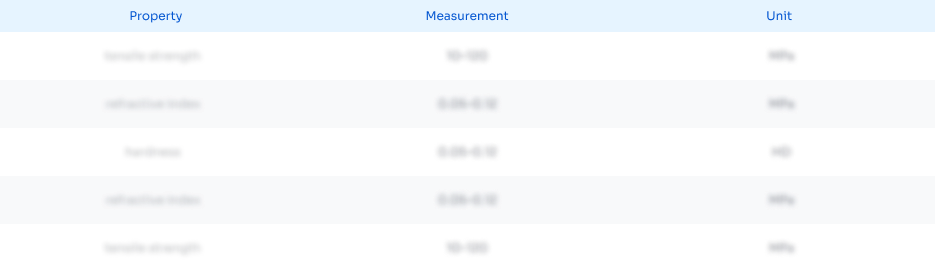
Abstract
Description
Claims
Application Information
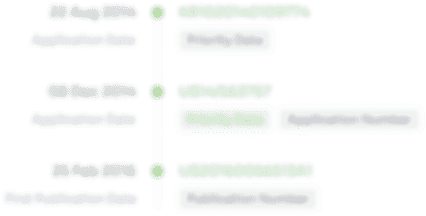
- R&D Engineer
- R&D Manager
- IP Professional
- Industry Leading Data Capabilities
- Powerful AI technology
- Patent DNA Extraction
Browse by: Latest US Patents, China's latest patents, Technical Efficacy Thesaurus, Application Domain, Technology Topic, Popular Technical Reports.
© 2024 PatSnap. All rights reserved.Legal|Privacy policy|Modern Slavery Act Transparency Statement|Sitemap|About US| Contact US: help@patsnap.com