Adversarial sample defense algorithm based on local disturbance
A technology against samples and local perturbations, applied in neural learning methods, biological neural network models, semantic analysis, etc., can solve problems such as migration of offensive and defensive algorithms to the text field
- Summary
- Abstract
- Description
- Claims
- Application Information
AI Technical Summary
Problems solved by technology
Method used
Image
Examples
Embodiment Construction
[0018] The present invention will be further described below in conjunction with the accompanying drawings. It should be noted here that the descriptions of these embodiments are only used to help understanding of the present invention, and are not intended to limit the present invention.
[0019] Such as figure 1 The algorithm is mainly divided into two important parts: cold start algorithm and local confrontation training.
[0020] The local perturbation adversarial training method is divided into the following stages:
[0021] 1. Adversarial training method
[0022] figure 2 Demonstrated a basic process of adversarial training, where the solid line part is a common step in conventional model training. For any model f(x), the purpose of training is to gradually confirm the value of θ in the model through sample x and label y . Each batch of training corpus x is put into the initialization model, and the loss value is obtained by comparing the model output value with th...
PUM
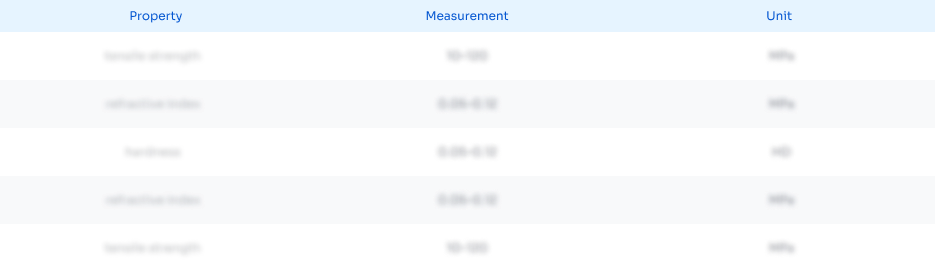
Abstract
Description
Claims
Application Information
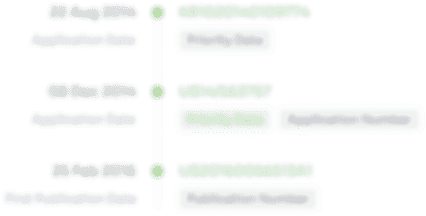
- Generate Ideas
- Intellectual Property
- Life Sciences
- Materials
- Tech Scout
- Unparalleled Data Quality
- Higher Quality Content
- 60% Fewer Hallucinations
Browse by: Latest US Patents, China's latest patents, Technical Efficacy Thesaurus, Application Domain, Technology Topic, Popular Technical Reports.
© 2025 PatSnap. All rights reserved.Legal|Privacy policy|Modern Slavery Act Transparency Statement|Sitemap|About US| Contact US: help@patsnap.com