Few-sample target detection method based on meta-feature and weight adjustment and network model
A technology of weight adjustment and target detection, which is applied in biological neural network models, neural learning methods, character and pattern recognition, etc., can solve problems such as difficult acquisition, poor generalization ability of overfitting, and less data, so as to improve target detection The effect of accuracy
- Summary
- Abstract
- Description
- Claims
- Application Information
AI Technical Summary
Problems solved by technology
Method used
Image
Examples
Embodiment Construction
[0041] In order to enable those skilled in the art to better understand the technical solutions of the present invention, the present invention will be further described in detail below in conjunction with the accompanying drawings.
[0042] Such as Figure 1-Figure 6 As shown, a few-shot target detection method based on meta-features and weight adjustment, the method includes the following steps:
[0043] S1. Build a detection network model and preprocess the input base class training images, new class training images and test images;
[0044] S2. Convolving the preprocessed base class training image into the meta-feature extraction module and the weight adjustment module in the detection network model to extract the corresponding meta-feature map and weight vector;
[0045] S3. Combine the meta feature maps and weight vectors extracted by respective convolutions to obtain the corresponding multidimensional feature maps, and then input the obtained multidimensional feature m...
PUM
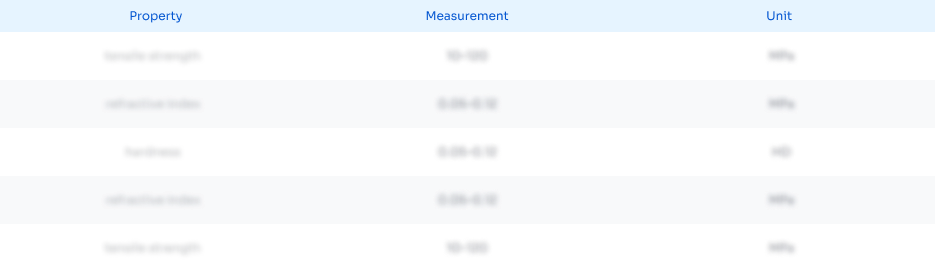
Abstract
Description
Claims
Application Information
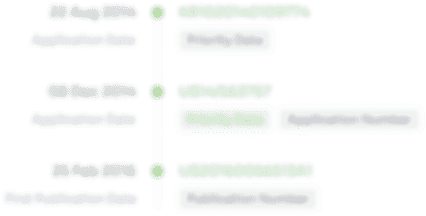
- R&D Engineer
- R&D Manager
- IP Professional
- Industry Leading Data Capabilities
- Powerful AI technology
- Patent DNA Extraction
Browse by: Latest US Patents, China's latest patents, Technical Efficacy Thesaurus, Application Domain, Technology Topic, Popular Technical Reports.
© 2024 PatSnap. All rights reserved.Legal|Privacy policy|Modern Slavery Act Transparency Statement|Sitemap|About US| Contact US: help@patsnap.com