Neural network-based improved RSG liver CT image interactive segmentation algorithm
A CT image and neural network technology, applied in the field of economic network, can solve the problems of low segmentation accuracy and weak stability of liver CT images, and achieve the effects of fine edge segmentation, improved stability, and simple operation.
- Summary
- Abstract
- Description
- Claims
- Application Information
AI Technical Summary
Problems solved by technology
Method used
Image
Examples
Embodiment Construction
[0037] For those skilled in the art, it is understandable that some well-known structures and descriptions thereof may be omitted in the drawings. The present invention will be further described below in conjunction with the accompanying drawings and implementation steps.
[0038] The present invention proposes an improved region growing algorithm based on a one-dimensional convolutional neural network to interactively segment liver CT images, and consider multiple information such as pixel gray values, spatial information, and different gradient values as growth rules through the neural network , which improves the stability of the region growing method and enhances the algorithm's ability to segment the complex structure of the edge.
[0039] figure 1 It is a flow chart of the method of the present invention, first image preprocessing, extracting the slices containing the liver in the CT image sequence set, using the window algorithm to convert the CT image into a graysca...
PUM
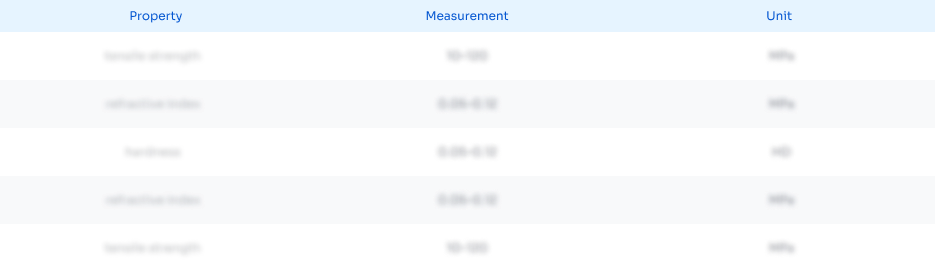
Abstract
Description
Claims
Application Information
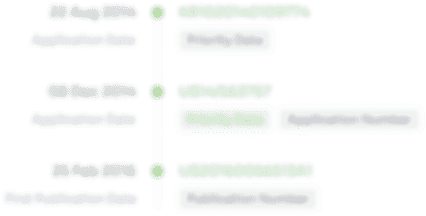
- R&D Engineer
- R&D Manager
- IP Professional
- Industry Leading Data Capabilities
- Powerful AI technology
- Patent DNA Extraction
Browse by: Latest US Patents, China's latest patents, Technical Efficacy Thesaurus, Application Domain, Technology Topic, Popular Technical Reports.
© 2024 PatSnap. All rights reserved.Legal|Privacy policy|Modern Slavery Act Transparency Statement|Sitemap|About US| Contact US: help@patsnap.com