Large aircraft point cloud model self-supervision semantic segmentation method based on deep learning
A point cloud model and semantic segmentation technology, applied in the fields of deep learning, computer vision and graphics, can solve problems such as low accuracy and low measurement efficiency, and achieve the effect of eliminating error accumulation, rational conception, and high global registration effect.
- Summary
- Abstract
- Description
- Claims
- Application Information
AI Technical Summary
Problems solved by technology
Method used
Image
Examples
Embodiment Construction
[0057] In order to better understand the technical content of the present invention, specific embodiments are described in conjunction with the accompanying drawings as follows.
[0058] Combine figure 1 , The present invention proposes a self-supervised semantic segmentation method for a large airplane point cloud model based on deep learning. The semantic segmentation method includes the following steps:
[0059] S1, using a laser tracker to collect large-scale aircraft point clouds from multiple stations to generate several sets of 3D point cloud data.
[0060] S2, construct a global measurement field, perform initial registration of several sets of 3D point cloud data based on the global measurement field, and then perform fine registration on the point cloud data after the initial registration based on graph optimization to obtain a complete aircraft point cloud model.
[0061] S3, self-supervised semantic segmentation of the complete aircraft point cloud model.
[0062] In the fie...
PUM
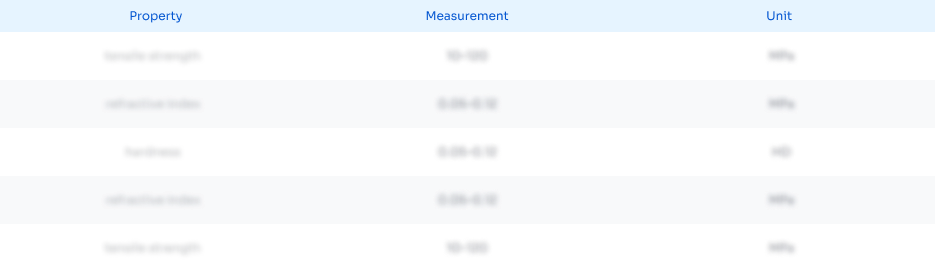
Abstract
Description
Claims
Application Information
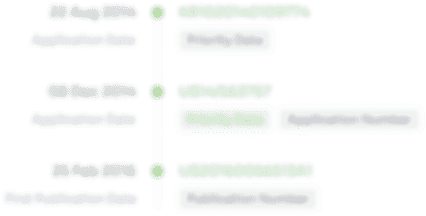
- R&D Engineer
- R&D Manager
- IP Professional
- Industry Leading Data Capabilities
- Powerful AI technology
- Patent DNA Extraction
Browse by: Latest US Patents, China's latest patents, Technical Efficacy Thesaurus, Application Domain, Technology Topic.
© 2024 PatSnap. All rights reserved.Legal|Privacy policy|Modern Slavery Act Transparency Statement|Sitemap