Deep network model compression training method based on generative adversarial neural network
A deep network and training method technology, which is applied in the field of deep network model compression, can solve problems such as decline, decline in accuracy and robustness of deep network models, loss of accuracy and robustness, etc., to achieve a stable training process and speed up model convergence. , the effect of improving the robustness
- Summary
- Abstract
- Description
- Claims
- Application Information
AI Technical Summary
Problems solved by technology
Method used
Image
Examples
Embodiment Construction
[0028] The technical solutions in the embodiments of the present invention will be clearly and completely described below in conjunction with the accompanying drawings in the embodiments of the present invention. Obviously, the described embodiments are only some of the embodiments of the present invention, not all of them. Based on the embodiments of the present invention, all other embodiments obtained by persons of ordinary skill in the art without creative work all belong to the protection scope of the present invention.
[0029] The deep network model compression training method based on the generated confrontational neural network provided by the present invention provides possible technical support for transplanting a large-scale deep network model to the FPGA platform. It mainly includes knowledge distillation of network structure information and training strategy of network model. The structural information of the network model is distilled by generating an adversaria...
PUM
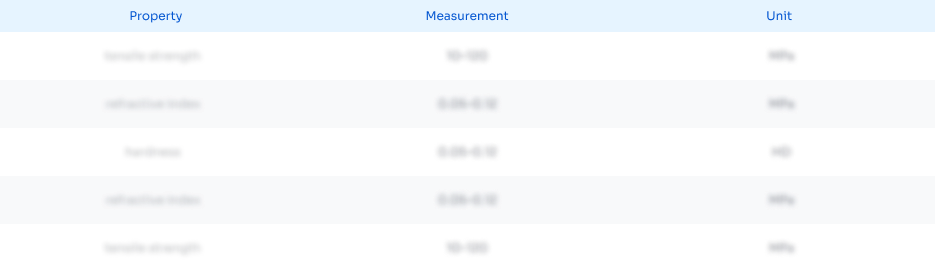
Abstract
Description
Claims
Application Information
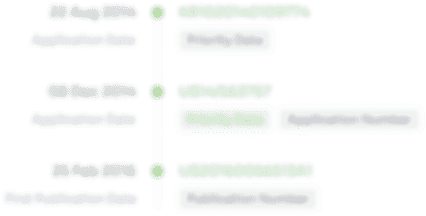
- R&D Engineer
- R&D Manager
- IP Professional
- Industry Leading Data Capabilities
- Powerful AI technology
- Patent DNA Extraction
Browse by: Latest US Patents, China's latest patents, Technical Efficacy Thesaurus, Application Domain, Technology Topic, Popular Technical Reports.
© 2024 PatSnap. All rights reserved.Legal|Privacy policy|Modern Slavery Act Transparency Statement|Sitemap|About US| Contact US: help@patsnap.com