Gesture recognition method based on convolutional neural network and 3D estimation
A technology of convolutional neural network and gesture recognition, which is applied in the field of gesture recognition based on convolutional neural network and 3D estimation, can solve the problems of limited model parameter scale, large uncertainty, and limitations of application scenarios, so as to improve reliability and the effect of precision
- Summary
- Abstract
- Description
- Claims
- Application Information
AI Technical Summary
Problems solved by technology
Method used
Image
Examples
Embodiment 1
[0055] Example 1: Reconstruction results of test samples after CBN-VAE network training
[0056] The data set used in this embodiment is a virtual data set (Rendered hand pose dataset, RHD) obtained by synthesizing a 3D animation character model and a natural background. 16 characters are randomly selected, and the samples of 31 actions performed by them are divided into training The data set contains 41258 320×320×3 images, denoted as RHD_train. The samples of the other 4 characters and the other 8 actions they perform are divided into a test data set, which contains 2728 images of 320×320×3, denoted as RHD_test.
[0057] The SegNet-base and SegNet-prop networks are trained, and the performance of the network is evaluated on the RHD_test test set. There is no other operation on the test data except for size adjustment. The evaluation results are shown in Table 5.
[0058] Table 5 RHD dataset performance comparison
[0059]
[0060] The evaluation results show that the Se...
Embodiment 2
[0061] Embodiment 2: train on the RHD_train data set and the STB_train data set respectively, then evaluate on the RHD_test data set and the STB_test data set respectively, and the evaluation results are shown in Table 6 and Image 6 . Table 6 shows the EPE differences between the two. The endpoint error in the table is in pixel units. At the same time, the AUC with an error threshold range of 0 to 30 is calculated. The arrow points to represent the change of performance with the value, and the upward indicates the value The bigger the better the performance.
[0062] Table 6 DetectNet performance evaluation
[0063] EPE mean(px)↓ EPE median(px)↓ AUC(0~30)↑ RHD_test 11.619 9.427 0.814 STB_test 8.823 8.164 0.917
[0064] According to the PCK curves of the DetectNet network under different endpoint error thresholds, it can be seen that when the error threshold is less than 15 pixels, the PCK value of the model increases rapidly; when the err...
Embodiment 3
[0065] Example 3: Evaluation of a complete 2D hand joint detection network
[0066]A complete hand joint detection network is formed by cascading the hand segmentation network SegNet-prop and the joint detection network DetectNet, which is denoted as PoseNet2D here. First, only train the hand segmentation network on RHD_train, that is, adopt the conclusion in the previous example. Then get a better DetectNet model on the Joint_train training set, and finally test the performance of the PoseNet2D network on the RHD_test, STB_test and Dexter data sets. Such as Figure 7 As shown, the solid line graph represents the PCK curves of the DetectNet model under the RHD_test test set and the STB_test test set respectively, and the dotted line graph represents the PCK curves of the PoseNet2D model under the RHD_train, STB_test and Dexter test sets respectively.
[0067] The results of the examples show that: the PoseNet2D model is suitable for RHD and STB datasets, and performs well, a...
PUM
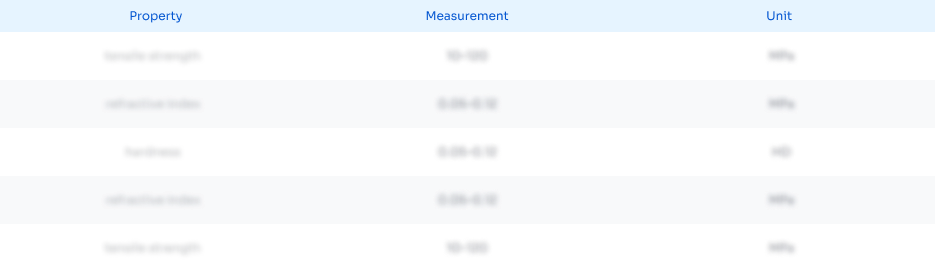
Abstract
Description
Claims
Application Information
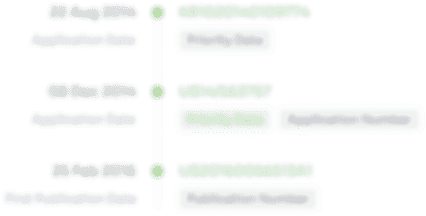
- R&D Engineer
- R&D Manager
- IP Professional
- Industry Leading Data Capabilities
- Powerful AI technology
- Patent DNA Extraction
Browse by: Latest US Patents, China's latest patents, Technical Efficacy Thesaurus, Application Domain, Technology Topic, Popular Technical Reports.
© 2024 PatSnap. All rights reserved.Legal|Privacy policy|Modern Slavery Act Transparency Statement|Sitemap|About US| Contact US: help@patsnap.com