A Rebar Detection Method Based on Deep Convolutional Neural Network
A neural network and deep convolution technology, applied in the field of steel bar detection based on deep convolutional neural network, can solve the problems of limited accuracy and robustness, difficult to put into practical use, etc., to improve geometric deformation ability and generalization Good, high detection accuracy
- Summary
- Abstract
- Description
- Claims
- Application Information
AI Technical Summary
Problems solved by technology
Method used
Image
Examples
Embodiment Construction
[0043] The present invention will be further described below in conjunction with specific examples.
[0044] Such as figure 1 As shown, the steel bar detection method based on deep convolutional neural network provided in this embodiment, its specific situation is as follows:
[0045] Step 1: Collect pictures of steel bars in the actual construction site, manually mark them, and divide them into training sets and test sets.
[0046] Step 2, perform data enhancement on the training set, including the following steps:
[0047] Step 2.1, carry out center cropping on the training set, cut off the surrounding edges, and only keep 85% or 90% or 95% of the center of the original image;
[0048] Step 2.2, perform multi-scale scaling on the cropped training set, such as zooming to 1000 pixels, 1400 pixels, 1600 pixels, etc. on the short side;
[0049] Step 2.3, horizontally and vertically flip the multi-scale zoomed image;
[0050] Step 2.4, rotate the multi-scale zoomed picture by...
PUM
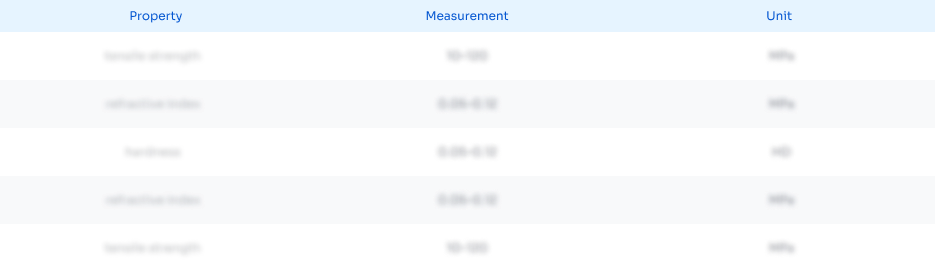
Abstract
Description
Claims
Application Information
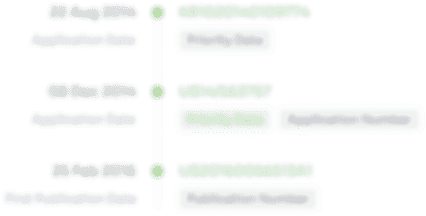
- R&D Engineer
- R&D Manager
- IP Professional
- Industry Leading Data Capabilities
- Powerful AI technology
- Patent DNA Extraction
Browse by: Latest US Patents, China's latest patents, Technical Efficacy Thesaurus, Application Domain, Technology Topic, Popular Technical Reports.
© 2024 PatSnap. All rights reserved.Legal|Privacy policy|Modern Slavery Act Transparency Statement|Sitemap|About US| Contact US: help@patsnap.com