Tool abrasion state identification method based on convolutional neural network and long-short-time memory neural network combined model
A convolutional neural network, long and short-term memory technology, used in manufacturing tools, metal processing mechanical parts, measuring/indicating equipment, etc., can solve problems such as the inability to reflect the timing of wear and tear, save the cost of selecting features, ensure accuracy and Accuracy, effect without expert experience
- Summary
- Abstract
- Description
- Claims
- Application Information
AI Technical Summary
Problems solved by technology
Method used
Image
Examples
Embodiment Construction
[0027] Below in conjunction with example and accompanying drawing, the present invention will be further described:
[0028] The present invention's tool wear state identification method based on the joint model of convolution and long-short-term memory neural network specifically includes the following technical steps:
[0029] Install the Kistler 9272 three-way dynamometer and the 1A302E three-way piezoelectric acceleration sensor on the fixture and the workpiece of the CNC machine tool, and then use constant cutting parameters to carry out side milling on the material under constant working conditions, and at the same time Connect the dynamometer to the Kistler5070A charge amplifier to collect three-way force signals and vibration acceleration signals. figure 2 To collect data for vibration signals, the machine tool used is XK714D Shaanxi Hanchuan Machine Tool, image 3 Acquire data for the force signal.
[0030] After collecting enough data, first perform data preproces...
PUM
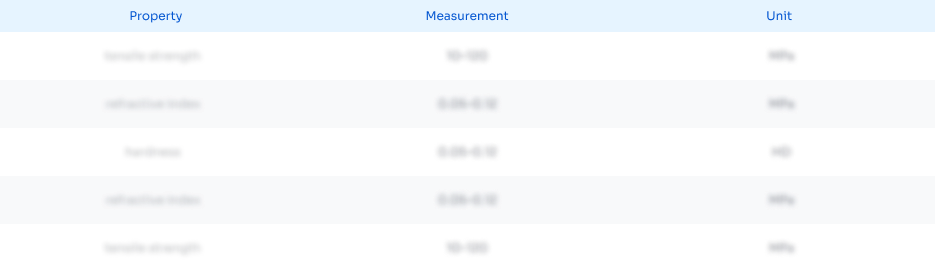
Abstract
Description
Claims
Application Information
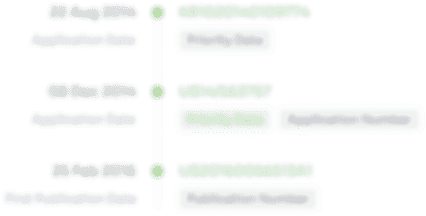
- R&D Engineer
- R&D Manager
- IP Professional
- Industry Leading Data Capabilities
- Powerful AI technology
- Patent DNA Extraction
Browse by: Latest US Patents, China's latest patents, Technical Efficacy Thesaurus, Application Domain, Technology Topic, Popular Technical Reports.
© 2024 PatSnap. All rights reserved.Legal|Privacy policy|Modern Slavery Act Transparency Statement|Sitemap|About US| Contact US: help@patsnap.com