A Method of Building Change Detection in Remote Sensing Imagery Based on Convolutional Neural Network
A convolutional neural network and change detection technology, applied in the field of deep learning, can solve problems such as the difficulty of complete registration of buildings and achieve strong robustness
- Summary
- Abstract
- Description
- Claims
- Application Information
AI Technical Summary
Problems solved by technology
Method used
Image
Examples
Embodiment
[0031] Embodiment: first according to figure 1We know that the overall framework of the present invention is composed of two parts: a building extraction network and a building change detection network. In order to train a network model, we need training data. The first step is to generate our sample library based on the existing aerial images and vector label data. Areas without label data coverage need to produce label data. To train the building extraction network, we organize the building classification dataset, and to train our proposed self-training building change detection network, we organize the building change detection simulation dataset. In order to further train the change detection network and further improve the accuracy of the change detection results, we sort out the real data set of building change detection (optional). All the data here are finally divided into small images of 512×512 for the convenience of inputting into the network.
[0032] The second...
PUM
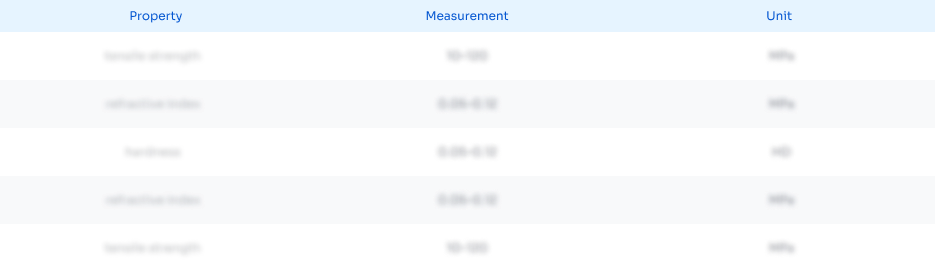
Abstract
Description
Claims
Application Information
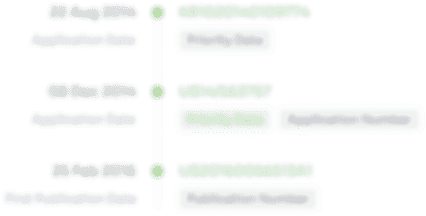
- R&D Engineer
- R&D Manager
- IP Professional
- Industry Leading Data Capabilities
- Powerful AI technology
- Patent DNA Extraction
Browse by: Latest US Patents, China's latest patents, Technical Efficacy Thesaurus, Application Domain, Technology Topic, Popular Technical Reports.
© 2024 PatSnap. All rights reserved.Legal|Privacy policy|Modern Slavery Act Transparency Statement|Sitemap|About US| Contact US: help@patsnap.com