Urban road congestion degree prediction method based on time sequence traffic events
A technology for road congestion and traffic incidents, applied in traffic flow detection, forecasting, traffic control systems, etc. Efficiency, avoidance of complex processes, effect of good applicability
- Summary
- Abstract
- Description
- Claims
- Application Information
AI Technical Summary
Problems solved by technology
Method used
Image
Examples
specific Embodiment
[0049] Step 1: Obtain more than 6,000 road network data through the urban road traffic information on the public security intranet and real-time congestion information uploaded by map software users, select 82 typical urban road sections, and extract time-series traffic events 30 minutes before each road section congestion event .
[0050] Step 2: The 3D CNN architecture based on Python is as follows: figure 2 As shown, it contains a hardwired layer, 3 convolutional layers, 2 pooling layers and a fully connected layer. The cube of each 3D convolution kernel convolution takes 7 consecutive frames, each frame size is 60×40, and the information of 5 channels is extracted through the first layer, which are: grayscale, gradient in x and y directions, x and y Directional optical flow; each layer of convolution kernel u×v×3 (u×v represents the spatial dimension, 3 represents the time dimension) respectively performs convolution operations on the information of the 5 channels; using...
PUM
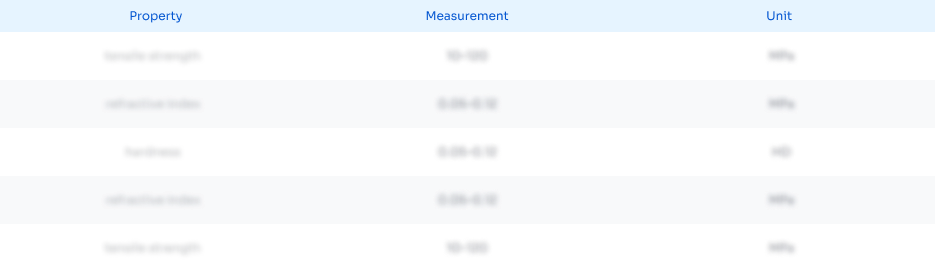
Abstract
Description
Claims
Application Information
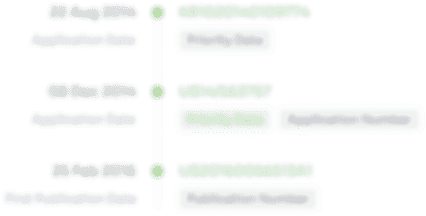
- R&D Engineer
- R&D Manager
- IP Professional
- Industry Leading Data Capabilities
- Powerful AI technology
- Patent DNA Extraction
Browse by: Latest US Patents, China's latest patents, Technical Efficacy Thesaurus, Application Domain, Technology Topic, Popular Technical Reports.
© 2024 PatSnap. All rights reserved.Legal|Privacy policy|Modern Slavery Act Transparency Statement|Sitemap|About US| Contact US: help@patsnap.com