Multi-scale target detection method based on deep convolutional neural network
A neural network and deep convolution technology, applied in the field of computer image processing, can solve the problems of low accuracy of small-scale objects, reduced robustness and effectiveness, loss of small-scale object features, etc.
- Summary
- Abstract
- Description
- Claims
- Application Information
AI Technical Summary
Problems solved by technology
Method used
Image
Examples
Embodiment Construction
[0068] The present invention will be further described below in conjunction with specific examples.
[0069] like figure 1 As shown, the multi-scale target detection method based on deep convolutional neural network provided in this embodiment, its specific situation is as follows:
[0070] Step 1. Obtain the highway video dataset, then obtain its video frames, manually mark them, and divide them into training datasets and verification datasets.
[0071] Step 2, converting the image and label data of the image dataset into the format required for training the deep convolutional neural network through preprocessing, including the following steps:
[0072] In step 2.1, the image in the dataset is scaled to a size of 768×1344 pixels in length and width, and the label data is also scaled to the corresponding size according to the corresponding ratio.
[0073] In step 2.2, in the scaled image, randomly crop the place containing the label to obtain a square image with a size of 76...
PUM
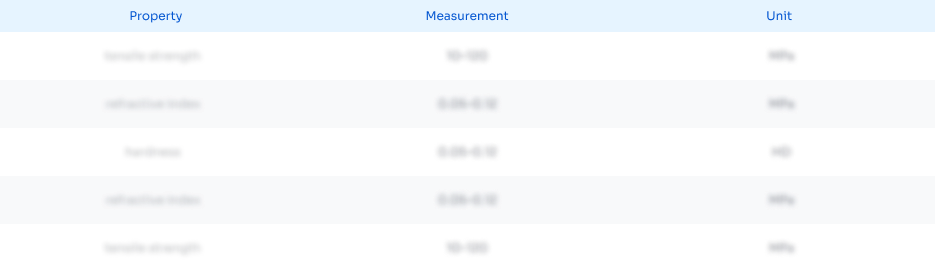
Abstract
Description
Claims
Application Information
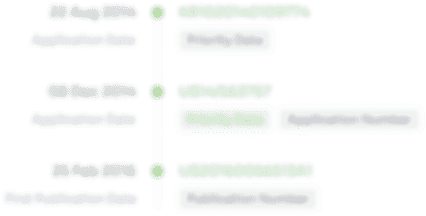
- R&D Engineer
- R&D Manager
- IP Professional
- Industry Leading Data Capabilities
- Powerful AI technology
- Patent DNA Extraction
Browse by: Latest US Patents, China's latest patents, Technical Efficacy Thesaurus, Application Domain, Technology Topic, Popular Technical Reports.
© 2024 PatSnap. All rights reserved.Legal|Privacy policy|Modern Slavery Act Transparency Statement|Sitemap|About US| Contact US: help@patsnap.com